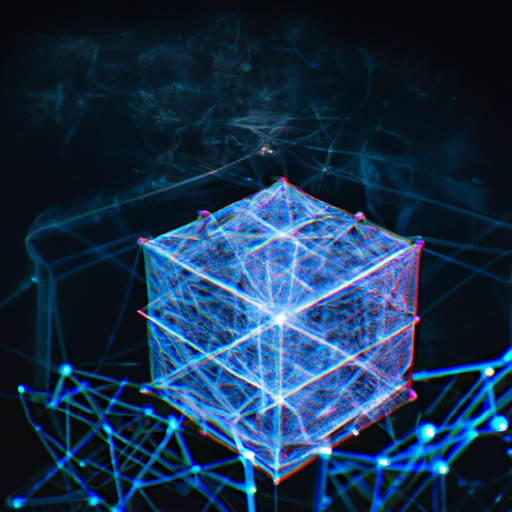
Resolves to expert consensus 2040
In this other market, HMYS issued a controversial resolution, then hid critical comments and offered to pay for positive reviews. You should perhaps consider that when deciding whether to put your mana in this market. https://manifold.markets/hmys/will-scott-win-the-book-review-cont?r=RGFuaWVsRmlsYW4
@DanielFilan I resolved it correctly, as I have all my other markets. People review bombed my markets because they lost money after trying to snipe the market on a technicality at the end. I asked people to review the market positively to correct for the people down-rating it out of spite.
For those who are unaware. The market was a book review contest hosted by Scott Alexander. A bunch of people would write reviews of books, then the readers of ACX would vote on those reviews, and the review that got the most number of votes would win. Scott himself entered the contest anonymously. I made a market on whether he would win. He did end up winning and getting the most votes. But he disqualified himself because he thought it would be improper for him to win his own contest. I decided to resolve the market YES, as what people were thinking about when reading the market for most of its existence was whether he would get the most votes.
eh, scott gets to decide who wins his book review contest. his post clearly declares winners and his post was not in the winner section, it was in the finalist section. it clearly says that it got the most voted, but didn't win.
Also none of the above is an excuse for paying for positive reviews and hiding critical comments, the things I complained about.
@jacksonpolack Doesn't get to decide how his writers interpret his words post-hoc unfortunately.
@vluzko My understanding is that a reason transformers are so influential is because they scale so well, so I would count that. It should be sorted out by the resolution criteria anyways though.
Reality is extremely geometric, so advanced AI is going to be geometric too. Either that can be done through hard-coding the geometric structure into the learning algorithm (aka geometric deep learning), or it can be done by letting the AI learn it from scratch.
I think hard-coding the geometric structure into the learning algorithm will give a huge boost to interpretability and alignment, because it gives a natural "interface" with which to work with. If the models learn it from scratch, the geometric structure they learn will be very black-boxy and hard to work with. Meanwhile, with geometric deep learning, it becomes trivial to e.g. inspect what the model states is at a given location, or similar.
@tailcalled Wait, looking it up, geometric deep learning is even broader than I thought. I thought it was just stuff like neural radiance fields etc., but apparently it also includes graph neural networks and stuff? At least for something like protein folding? Not sure about the specifics, but the more different things fall under the "geometric deep learning" label, the more likely it seems to me to become as influential an idea as transformers.
@tailcalled transformers is a special case of GNNs which is a special case of geometric DL, it's gonna be big bro, geomtric DL is the category theory of DL
@tailcalled CNNs is also a speical case of geometric DL. It kind of feels like many of the breakthroughs in DL is just the result of building in some geometric priors into the network, like translation invariance, permutation invariance, invariance to isomorphic graphs, etc.
@tailcalled This is largely due to it decreasing the effect of the cure of dimensionality since the input space gets largely diminished by all of the invariants (because multiple data instances induce the same representation in the network due to the invariants)
In order for this to resolves YES, 'geometric deep learning' or an analogous term or field should be considered as influential as transformers in 2040. What I don't want is for some new architecture or thing to take off, and then someone to say "well technically this is geometric deep learning because it can be described as a graph"
Consider, from the wikipedia article, "Convolutional neural networks, in the context of computer vision, can be seen as a GNN applied to graphs structured as grids of pixels. Transformers, in the context of natural language processing, can be seen as GNNs applied to complete graphs whose nodes are words in a sentence." Surely this doesn't already resolve YES because transformers and CNNs are big?
@jacksonpolack I don't think so, in the same sense that category theory would be considered influential idependent of the important ideas it generlizes, i.e. the importance doesn't stem from the fact that any single idea it generalizes is important, but from the fact that it generalizes many important ideas.
Analogously - I might say that hypergraph deep learning theory is extremely influential, because graph neural networks are also hypergraph neural networks. Surely this isn't what anyone means, or how anyone would interpret that statement.
This should only resolves YES if geometric deep learning is seen by the practitioners of deep learning to be influential, how they interpret geometric deep learning. Not merely YES if future neural networks are interpretable as graphs (as they will be becauase they already are)
@jacksonpolack I think the appeal to expert consensus solves this issue. I don't think experts would consider an idea influential just because you can reinterpret already existing concepts using that idea (unless it produces novel insight) or because you can argue that already existing concepts already are instantiations of that idea.