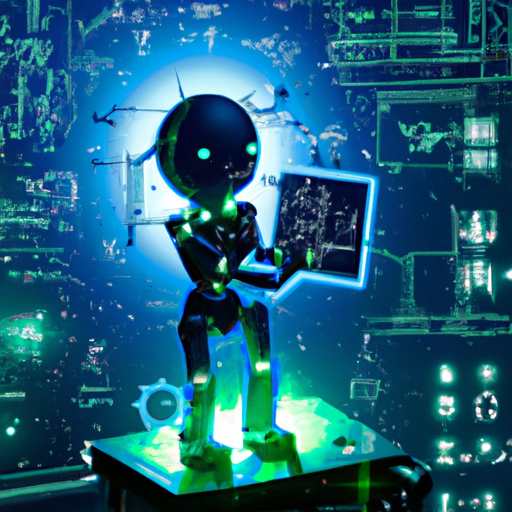
Will a state of the art model on Hendrycks' MATH be trained for more FLOP on RL than it is on LM objectives? A purely RL model counts as well of course.
RL encompasses anything involving online learning or expert iteration-like etc. If this ends up being difficult to call because of some breakthrough in decision transformer style conditional imitation learning (ie something between rl and LMs), I will probably cancel the market as ambiguous.
When models approach 100% acc on MATH, a similar successor natural language math dataset will be used instead.
https://deepmind.google/discover/blog/ai-solves-imo-problems-at-silver-medal-level/
I'd guess this took something like 1-10 trillion tokens worth of FLOPs.
@Thomas42 That’s a bit tricky, but I’d say kl penalties from base LM should just be counted as part of the RL compute. That’s not an LM loss anyway.
If this question ends up hinging on some edge case like a method which does continued LM training during RL, and the relative compute contributions are unclear Ill probably resolve N/A.
I think the first question one should ask is will anything overtake LMs. The probability that one specific technology would be the one doing the overtaking should then be below that base probability. I place the first probability at around 50%, so I am comfortable betting against this at the current price.
@vluzko I wanted to exclude decision transformer type stuff. Maybe it would be more fair to have titled the question 'Will online learning overtake offline learning for LMs on Math...', but I went for something more eye-catching.
I'm interested in this because I'm interested in the data shortage in terms of imitation learning data available. I also think offline learning has different safety properties.