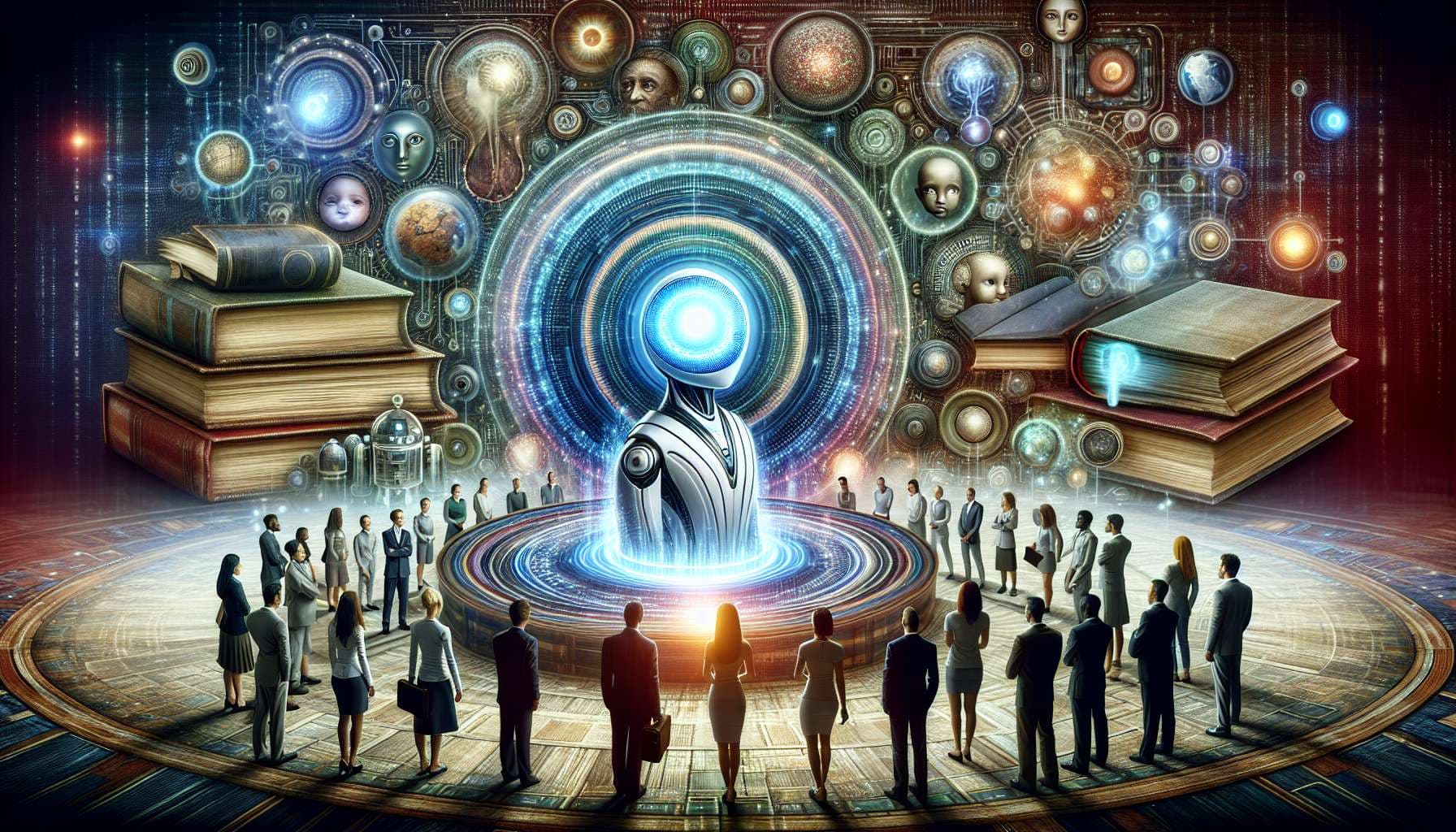
Resolve's when the o3 model is usable in the ChatGPT app (mobile or web) for people with a Plus level subscription (which at the time of writing is $20/month).
Update 2025-02-12 (PST) (AI summary of creator comment): Additional Resolution Criteria:
Based on Sam Altman's tweet, o3 will not be released standalone.
This market resolves to NA.
🏅 Top traders
# | Name | Total profit |
---|---|---|
1 | Ṁ336 | |
2 | Ṁ158 | |
3 | Ṁ121 | |
4 | Ṁ117 | |
5 | Ṁ71 |
People are also trading
@Bayesian no I think you got it exactly right, thanks so much!
can you also please do for the sister market?
https://manifold.markets/gallerdude/when-will-openais-o3-model-release-N9U9PhLzEz?play=true
Sam Altman has tweeted that o3 will not be released standalone, this market resolves to NA. Follow up market for GPT-4.5 Orion here:
https://manifold.markets/gallerdude/when-will-gpt45-orion-be-released
@gallerdude isn't "before march" going to be the market that supplies the most informative content though?
@MalachiteEagle looks like people are betting that o3 will require a ChatGPT Pro subscription, at least initially (I disagree with those people)
@JoshYou yeah seems odd that they would only limit it to a tiny percentage of their users. The whole point of the new double scaling law paradigm is that if you continue the RL training then you get an improved model for the same inference-time compute cost. They can entirely release o3 with fairly low compute settings and just call that "o3", and have different names for higher compute tiers like "o3-pro" etc
@4fa oh, I see what you mean. I guess this’ll have to resolve in 2027. If it doesn’t resolve by then, it’s a NO.