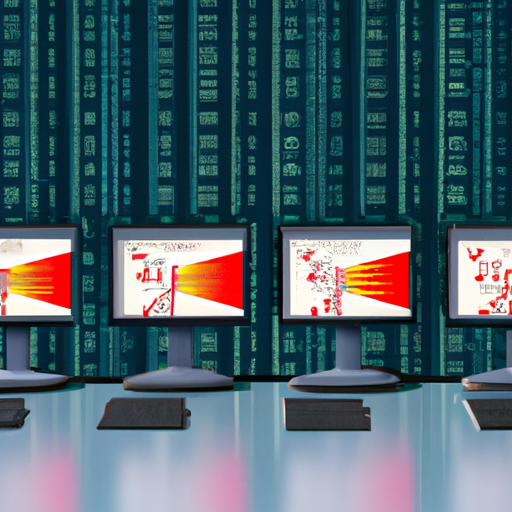
When GPT5 comes out, plot a graph of its capabilities according to all quantifiable metrics, and chart that against the cost to train it and operate it. If it's clearly getting more expensive faster than it's getting better, or if the increases in capabilities are a significant slowdown from previous jumps (GPT2 to GP3 to GPT4) this resolves YES.
CLARIFICATION: RE: the last line about capabilities, what I meant was, "if there is a significant slowdown in capabilities from this generation to the next sufficient to mean the cost curve still shows diminishing returns," which in hindsight is redundant. I spun off a separate market for those who want to bet on JUST whether the capabilities jump will be less than we've seen before, regardless of cost curve: https://manifold.markets/LarsDoucet/will-the-performance-jump-from-gpt4
If there's no CLEAR signs or it's ambiguous, this resolves NO.
One guess is that they created a big (50-100T tokens) synthetic dataset with gpt-4 and did some scaled up training runs with this in the pretraining mix, and it didn't work super well. The leaks we're hearing now are related to the checkpoints produced downstream of this effort (+ with post-training).
Good chance they already have a parallel effort ongoing where o1 is used to create long-context token sequences with higher quality reasoning, and will include this in the pretraining mix to boost performance in the following scaled up training runs.
Now that OpenAI has announced that they will be doing rolling releases instead of one big GPT-5 drop, how will this resolve? https://youtu.be/1NAmLp5i4Ps
@jonsimon If there’s not ever a GPT5 release, I might have to resolve it N/A, unless I can find something they release that is really obviously substantially similar in a way nearly everyone would agree to.
Here's a market for those who want to bet on the related (but very different) question of whether the jump from GPT4->GPT5 will be less than the jump from GPT3->GPT4:
https://manifold.markets/LarsDoucet/will-the-performance-jump-from-gpt4
sublinear scaling is already true of all llms (meaning we have to grow parameters, dataset, compute exponentially to achieve a linear improvement in model performance, which is the definition of diminishing returns) https://manifold.markets/LarsDoucet/will-gpt5-show-clear-signs-of-dimin
@ConnorMcCormick Right, this market is about whether GPT5 will find some way around that currently observed trend.
@LarsDoucet In other words, we would have to see one of these curves start bending downward in order to resolve NO
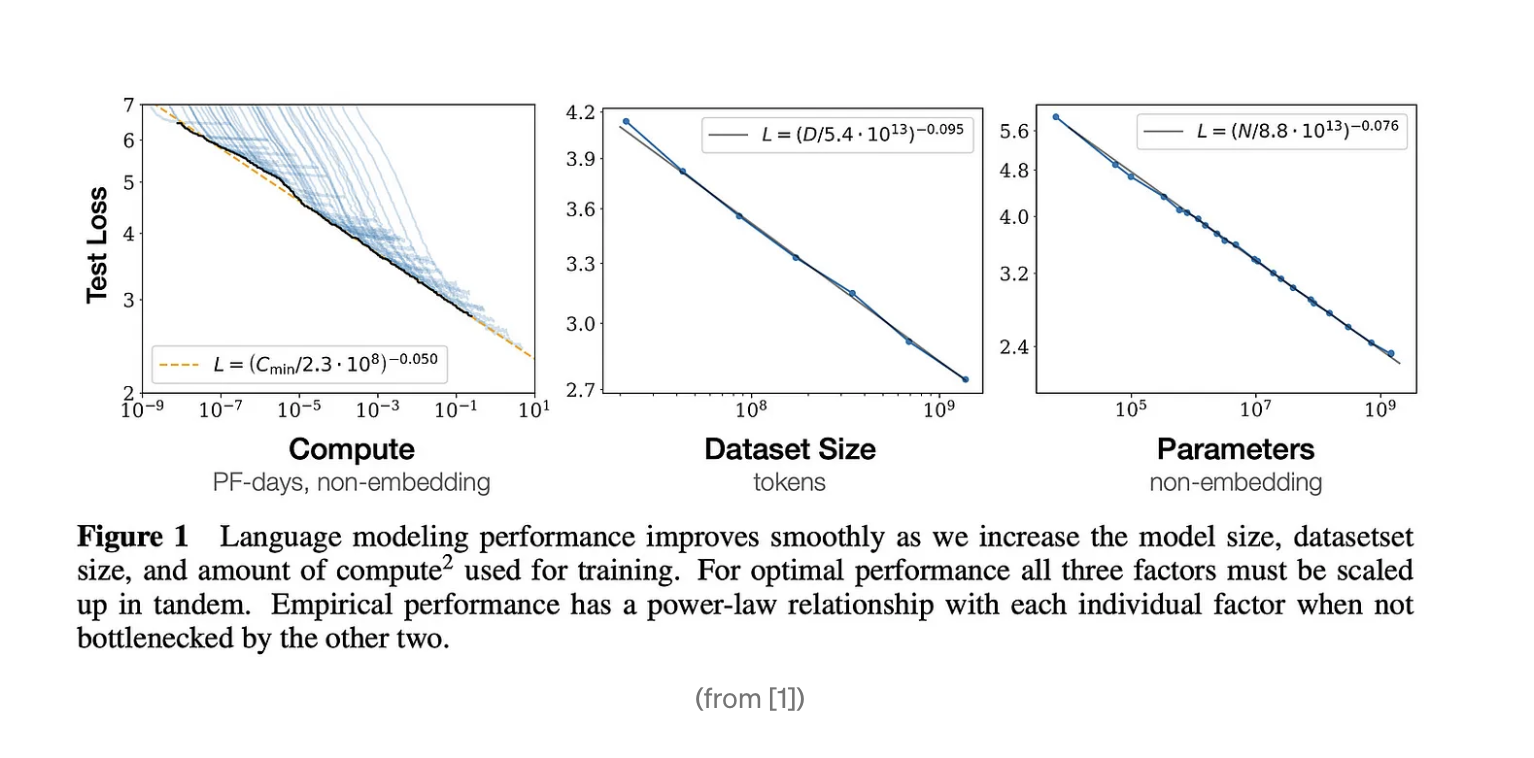
https://towardsdatascience.com/language-model-scaling-laws-and-gpt-3-5cdc034e67bb
@ConnorMcCormick Yes, we would need to see a break with the established pattern. The most likely cause in my mind would be some kind of paradigm shift or other fundamental breakthrough, but regardless of the cause you'd have to substantially break free from the established pattern of "it keeps costing more and more to get the next marginal unit of performance."
I suspect that people are overfocusing on the "capabilities increase isn't a significant jump" part and not the "getting more expensive part". GPT-5 very likely will be a significant jump in capabilities, it's just that that will require a correspondingly giant jump in cost. We will definitely get algorithmic efficiency improvements in the mean time, but it would be very surprising if we got efficiency improvements that make scaling not exponential in compute requirements. And if we do get that it's almost certainly AGI time and this market won't matter.
2 prices of evidence in favor:
Figure 2 from the GPT-4 paper, showing the training loss near-flatlining
Sam Altman saying that new breakthroughs would be needed for AGI in his Lex Fridman interview
Could we get clarification on if a continuation of a logarithmic trend in model size to training loss fulfills this criteria? I would say a logarithmic loss training curve is almost definitionally "diminishing returns", but it depends on whether you think training loss corresponds linearly with "capabilities" which is quite open to interpretation.
@Weepinbell See below:
> ASKED: Okay so more clarification: if scaling laws remain the same that resolves YES? What if some of the best predictions of the coefficients change, but the overall form of scaling laws (exponential costs for linear gains) stays the same?
> ANSWERED: If the overall form of scaling laws stays the same, I don't care so much about what the coefficients are, and this still resolves YES.
@LarsDoucet The spirit of the question is whether GPT5 will continue down the observed scaling laws we've already seen (which seem to indicate diminishing returns), or whether it finds a new paradigm and escapes those limitations.
In terms of model size vs training loss, the market as stated is looking at "all quantifiable metrics" -- so capabilities in general, but that cashes out to benchmarks, essentially. So it's not just training loss, it's all capabilities benchmarks (all the usual eval scores but also SAT score, Bar exam score, etc)
It's already the case that an OOM more compute does not get anything close to an OOM improvement (performance increase is closer to log of compute increase), this has been known since at least the first scaling laws paper. Are you just asking if that trend will continue? Or if we will see something significantly lower than predicted by scaling laws?
@LarsDoucet Okay so more clarification: if scaling laws remain the same that resolves YES? What if some of the best predictions of the coefficients change, but the overall form of scaling laws (exponential costs for linear gains) stays the same?
@vluzko If the overall form of scaling laws stays the same, I don't care so much about what the coefficients are, and this still resolves YES.