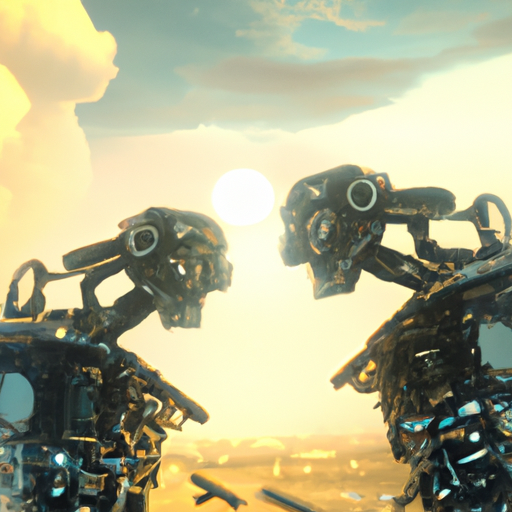
Resolves 3 months after Bard is switched from PaLM 2 to Gemini. (Resolves NA if it isn't switched to Gemini for some reason)
Unless I'm given a very compelling reason, resolution will be based on https://github.com/FranxYao/chain-of-thought-hub
Specifically - if 3 months after Gemini is released it has at least 3 scores better than GPT-4, and has at least twice as many scores better than GPT-4 than worse, this market resolves YES. Otherwise, assuming no major contention, resolves NO. If not enough benchmarks are available on this repo I will try to find something similar.
Related questions
🏅 Top traders
# | Name | Total profit |
---|---|---|
1 | Ṁ3,757 | |
2 | Ṁ2,323 | |
3 | Ṁ1,357 | |
4 | Ṁ957 | |
5 | Ṁ948 |
The market will close soon, so now is the time to fill in the gaps in the chain-of-thought hub table https://github.com/FranxYao/chain-of-thought-hub.
Specifically these numbers could affect the resolution:
GSM8K accuracy for Gemini Ultra
C-eval accuracy for Gemini Ultra
BBH accuracy for GPT-4
In order to affect the resolution, the number needs to be accepted into the repo. If when the market closes there's an open PR for one of these and it would change the resolution, I'll wait a few days for it to get merged before resolving.
@SimranRahman Right, but that's an updated version (not released yet if I understand correctly), so does not affect this market which is about the state of affairs at release.
@chrisjbillington Yes. But more specifically - I will resolve the market based on Gemini Ultra 1.0. That's the treatment that's most consistent with how I said I'd treat new versions of gpt-4
Microsoft has new benchmarks out that seem to be a better apples-apples comparison. Google did more rigorous prompt engineering that had been standard in benchmarks in the past.
@WillSorenson Microsoft and Google are on a competition to see who can out-cherrypick the other.
@WillSorenson cool 🙂 honestly if gemini isn't even as good as gpt-4 that's very good news for humanity
@WillSorenson the benchmark hacking is getting a bit comical at this poit. I guess Microsoft is implying "Google got its 90.04% score the same way. We're just being honest about it."
What stood out to me is the re-tested zero-shot scores, with GPT4 now consistently ahead of Gemini Ultra....
Looks like Gemini Ultra scores are on Chain of thought hub - there are three tests in common with GPT-4, two surpassing it and one below (MMLU is below I'm assuming because of taking the 5-shot results). Technically this would mean resolving NO unless something changes until March 6th, but it's definitely close (Doesn't fulfill the "3 scores better" requirement).
@YoavTzfati I'm invested on yes and agree that resolving No based on your explanation is above board.
2k lim no at 70 (50 seems ... low though? like if gemini has data contamination that affects benchmarks this still resolves yes)
Looks like the Gemini Ultra demo was mostly fake https://twitter.com/parmy/status/1732811357068615969
I love that ‘above 90%’ turns out to be exactly 90.04%, whereas human expert is 89.8%, prior SOTA was 86.4%. Chef’s kiss, 10/10, no notes. I mean, what a coincidence, that is not suspicious at all and no one was benchmark gaming that, no way.
@JonasVollmer Their benchmarks were industry standards like BBH and MMLU, done the same amount of shot on both models. I don't see how that could be cherry picked.
@ShadowyZephyr You could fine-tune your model in such a way that it does well on MMLU, then selectively only report further benchmarks if it does better on them, and omit the ones it does worse on. You can run the benchmarks multiple times (different temperature etc.) and only report the best runs for your own model, and only the worst ones for competitors.
@JonasVollmer The whole point of the MMLU is that it’s very generalized and covers a broad range of useful skills for an AI assistant. If it is “fine-tuned” to do well on MMLU, then it will be able to do normal tasks too, making it better. All the benchmarks listed are well known standards as well.
@ShadowyZephyr Broad and generalized benchmarks are actually one of the major problems with LLMs. They tell you nothing meaningful about specific and narrow use cases. This is particularly a problem with high stakes use cases.
@BTE These models are made to be broad though, not cover specific narrow use cases with the highest performance. There are separate models that do those things.