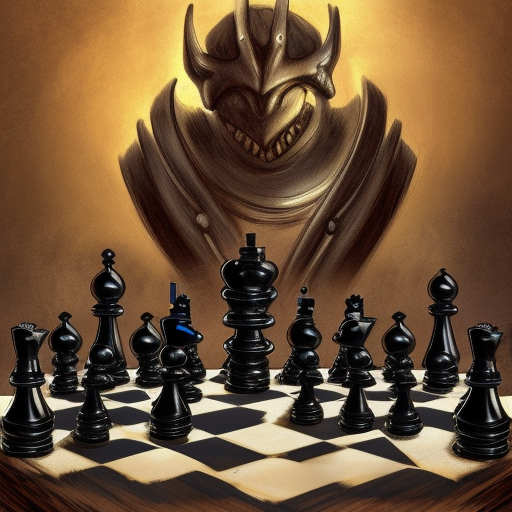
We're playing as black, against lichess's level 3 stockfish bot as white. Will we win?
Resolves YES if we get a checkmate / lichess resigns, or NO if we're mated by lichess, or PROB at 50% if it's a draw, or N/A if I'm unable to continue for some reason.
Link to the game: https://lichess.org/sLmZPb9nTcN
The current move is
Move History
g3 d5
Bg2 e5
Nf3 Bd6
c3 Nf6
O-O d4
Ng5 dxc3
bxc3 h6
Ne4 Nxe4
Qb3 Nc5
Qc2 e4
Bxe4 Nxe4
Qb3 Bf5
Na3 Qc8
Nc2 Be5
Qd5 Nd7
Ba3 c6
Qd3 Nxg3
fxg3 Bxd3
exd3 Qb8
Nd4 Bxg3
Nf3 ...
I'll post a separate market for each turn, that will close daily at midnight PST. Anyone who holds YES on this market can vote for a move in the comments.
Last time manifold played chess (https://manifold.markets/group/manifold-plays-chess), we lost -- someone bought a bunch of NO and then bid up bad moves. This is an experiment to see if that can be avoided with good market structure.
Related questions
🏅 Top traders
# | Name | Total profit |
---|---|---|
1 | Ṁ6,444 | |
2 | Ṁ1,972 | |
3 | Ṁ563 | |
4 | Ṁ351 | |
5 | Ṁ61 |
Testing this, might work?
https://manifold.markets/deagol/quadratic-funding-whats-a-good-open?r=ZGVhZ29s
Post describing the state of manifold chess is up at https://manifold.markets/post/manifold-plays-chess-2-retrospectiv
Please let me know if you spot anything you think should be added, removed, or changed.
@Jason giving up the queen or a piece, or even just a couple pawns, would also achieve a loss against mid-level opponents. So many bad moves to pick. There’s orders of magnitude more paths to a loss than there is to a win against a decent adversary.
@ZZZZZZ thats a good argument. I now think I would allow a Resign move in the first 5 moves of the game in my proposal.
@deagol The crowd, at least with engine assistance, is probably about 2500 elo better than Stockfish level 3 (1400 lichess rating = maybe 1100 FIDE?). I'd vote for full-strength SF even at queen odds, which is generally the worst one arbitrary move would get unless a forced mate is on the board AND SF 3 picks it up. You could also strategize to minimize the effect of one arbitrary move (e.g., trade queens early). With resign, the game isn't much different than "can someone get resign chosen by move X" which I find less Theoretically and practically interesting
@Jason Level 3 Stockfish is a useful test case, but in the ideal the ideal a prediction market should be at least as good as any other information-gathering system. I'm not very interested in market structures that restrict the available moves, or that perform much worse than a publicly available oracle. If the market chooses the worst available move from a short list of good options, IMO that's still a failure, even if we win the game.
"Resign" as an option makes market failures much more obvious and only marginally more likely. For example, see @jfjurchen's queen blunder in round 1 (https://manifold.markets/AlexLiesman/manifold-play-chess-11-qf3-bb7-12)
Here is my proposal for v3:
Per move, there will be 3 markets.
Fjrst, a free response market. The free response market wjll resolve to the move that manjfold wjll play. For each response, the average probabjljty jn the last hour before close js measured. Then two moves wjll be randomly drawn, wjth wejght proportjonal to those market probabjljtjes.
Then for each of the two candidate moves, a conditional market is created.
The markets for the candidate move are of the form "Will white win if we play move X?". For both conditional markets, we measure the average probability in the last hour of the market. The move with higher probability wins and gets chosen. The conditional market that does not get chosen gets resolved N/A. The conditional market for the move that gets chosen resolves to the score one move later (i.e. is determined by the score (by default average probability in the last hour) of the winning move). If there is no later move, the score for this purpose is 1.0 - #moves x 0.0005 if white win, 0.5 - moves x 0.0002 if its a draw, or 0.0 + moves x 0.0005 if we lose (this is to encourage a shorter game).
If the probabilities for the conditional moves stagnate, I plan to introduce some kind of leverage, so that predictors can predict in a wider range. An example for what I mean by leverage: if the score stagnates around 0.65, we could map 0.6 to 10%, and 0.7 to 90%, and interpolate for the rest.
Any comments, advice, feedback? Any ways to make sure I have good participation?
@harfe appareantly, I hit the wrong keys for a while. apologies. Also, I am leaning towards playing against stockfish 4 this time.
@harfe I can see a couple likely flaws with this:
The free response market to pick the two candidate moves is basically a vote-by-mana and is easily exploitable. It is like manifold plays chess #1 except now with two moves instead of 1 - an exploiter can simply buy their two chosen moves up to 50% each, it's not really that different I think.
Measuring the win probability only 1 move later on the next win probability market can also be easily manipulated. It's very close to a self-resolving market, just with two markets instead of one.
I think it's probably better for the move selection to be as far from a mana-vote as possible. If it was simply people proposing one move each and then using RNG to randomly select some number of them, that would actually work better I think.
And for the conditional markets, I would make them check the win probability more moves out, and possibly use an objective metric (could be something simple like material values, or a weak stockfish evaluation), similar to the ideas mentioned in https://manifold.markets/jack/will-someone-run-a-manifold-plays-c
@jack why resolve to win probability more moves out? The capital will just be locked up longer.
I'm opposed to using an engine in our Market design. And material count is the wrong metric in my opinion.
@harfe Material count is obviously wrong but it's a heuristic. My claim is that using a prediction market metric that can be manipulated is likely to do far worse than using a heuristic based on the board state.
Looking more moves out is mainly important when using heuristics; if using the future market win probability then it doesn't make much difference.
@harfe wouldn’t an attacker profit from buying NO’s on winning moves conditional markets, forcing them to close at progressively lower and lower prices (thus forcing resolution of the previous ones at profit), and finally rigging up “resign” as the winning choice for the last move? Heck, why wouldn’t they be able to do it on the very first move? Capability for taking over the two conditional markets is clear, especially if attacker knows one of them is risk free (N/A).
For example, on first move free choice, bet huge for both “d4” and “resign” so both get picked, then on conditional markets bet “resign” down to a low x%, and on “d4” conditional bet any amount so it closes lower than x. Manifold resigns, attacker profits in free choice move picker, the “resign” conditional, the main game market (assuming there is one), and N/A on the d4 conditional.
@jack just to clarify, that heuristic would be used for the score at which the earlier conditionals get resolved (as PROB), not to pick move candidates, right?
@deagol first I think I would not allow resign as a move.
I don't think your example works. Someone else could make a huge profit by buying up d4 slightly above x%.
@harfe why wouldn’t the attacker throw all their might on a risk free N/A market? Does the average participant know they must collectively fend off well over 100k in mana?
@harfe ok if resign not allowed then progressively lower scores and end up with a bad move that achieves the same. Doesn’t stop the attack.
@harfe The fundamental problem is that the reasoning/prediction is very circular - at a high level, the markets are predicting whether the mechanism will win or lose which is mostly driven by how well the markets work and what the market participants are incentivized to do. At a low level, the conditional markets are predicting the result of another market, which is easy to manipulate.
That's why I propose making the markets measure something explicit about the board state, which cuts the circularity.
Yeah, resign isn't special, you can replace it with any move that's a bad blunder.
@harfe I see, I guess I am approaching this from the perspective of trying to design futarchical market mechanisms that actually work.
@deagol These sorts of heuristics work well enough for simple chess engines, why wouldn't it work for Manifold? The bar we have right now isn't playing chess really well, it's being able to beat stockfish-3.
@jack I’m not an expert but I’m pretty sure even the simplest engines do way more than material count, and even for the material aspect, if the valuation is jumping +/- a pawn or two per move (because exchanging pawns or pieces), are programmed to look further ahead until it settles (exchanging material is over).
SFL3 seemed to me randomly making obvious blunders that a beginner would know are bad. Way worse than the simplest machines I played with back in the 80s.
@deagol Yeah, I've built simple chess engines in the past and they use some board evaluation function based on material, mobility, development, etc. https://www.chessprogramming.org/Evaluation. Any such function would work for my proposal, I just picked the simplest and dumbest one as an example.
@jack just to clarify, that heuristic would be used for the score at which the earlier conditionals get resolved (as PROB), not to pick move candidates, right?
Right.
@jack So in a futarchical mechanism you wouldn’t have the benefit of an engine? You define some measures of wellbeing, bet on markets for policies presumably improving those measures, wait a few years (or decades), and resolve the markets based on the observed changes in that measure. Did I get it right?
@deagol Yeah, that is the most common proposal I've seen. Of course if you have the equivalent of a strong chess engine you don't need markets, you'd just use the engine. But you could think of a weak engine as analogous to a program/person/organization that comes up with policy proposals and estimates how good they are - which the market would presumably take into account just by people looking at the info and trading accordingly.
@deagol regarding your example: the attacker needs to prop up the Resign market to x%, knowing that it will resolve negatively. Easy risk-free profit for others. But it is not obvious that people will the free money. Manifold markets are not efficient in my experience.
@harfe right, I wasn’t thinking straight, my bad (was focusing too much on fighting to hold down d4). So instead, they pick two bad moves in free choice? I guess then everyone joins the attacker’s side?
@jack I don't like the heuristic evaluations -- I think they're either vulnerable to goodharting or require lots of nuanced design work -- but I can see how it's the only practical way of getting a market to work, especially in the near term.
I would attack @harfe's proposed structure by buying up two equally bad moves at the "which move will we play?" free response stage. I like the structure in that if there's at least one good move chosen it will probably win, but I'm not sure 2 is enough to guarantee that.
See @jfjurchen 's comments at https://manifold.markets/citrinitas/will-white-win-in-alexliesmans-mani#jsUqPrcOMkMTsb3kcIXc for a description of why it's a good idea to try to resolve the conditional policy markets early. Basically: having those markets last until the end requires participants to lock up a large amount of mana for each move, which is hard on honest participants and creates a bigger bounty for manipulators.
If/when I run another game, I want to try my "reward function + exponential discounting" concept. I think it's probably pretty resistant to manipulation, doesn't require heuristics, and time-preference can be tuned. The downside is it basically requires a lot of bot action, but I'm working on writing that.
@deagol Ugh, everything is so scattered, this is why I want to do the write-up but I'm a slow writer.
I posted in comment on @jack's other market at https://manifold.markets/jack/will-someone-run-a-manifold-plays-c#7VmIXnFq4CbRfHliMhX5
To describe it in a less clunky way: the winning policy market resolves to PROB, based on the inner product of the evaluation market and an exponential decay function. Truncate after a few days when the decay gets small enough.
For example: say the "Will we win?" market is at 50%, 60%, 20%, 90%, 95% over five days. Then the move that won at the beginning first day will resolve to PROB at
(1/2 * 50) + (1/4 * 60) + (1/8 * 20) + (1/16 * 90) + (1/32 * 95)
... except the 1/2 term can be tuned to favor the long term more or less, and I'd want to sample continuously instead of discretely to prevent manipulation
@citrinitas ok wow think I was with you until you said convolution, but I probably got the gist of it. Perhaps this is the kind of simple function you’re after?
@deagol 😆 yeah. I'd say "If only we had LaTeX" except I'm no good at that either. I'll make sure it looks good in python.
Also, way down the line: once we beat chess I want to try Onitama, another two-player perfect information turn-based game. There are engines but not nearly as accessible as stockfish, which I think will make for a more entertaining market
@citrinitas wait but the evaluation market changes it’s value almost continuously by the participants betting instead of on every move, at a much higher quantization level (at every bet made) than the moves chosen (daily or every two days), so I’m not sure how the smoothing formula you described (and in my link) would work?
@deagol It's an inner product, not convolution -- the goal is weighting, not smoothing. The exponential function is just a fancy way of taking a weighted average of the evaluation market that cuts out after a few days.
We want to reward moves that are good and punish moves that are bad. But there's only one evaluation market and its value depends on the entire sequence of moves, so how do we use that to decide which moves are good and which are bad? With exponential decay, we say that a move affects the evaluation function strongly immediately after it's played, and more weakly as time goes on. If we choose γ =1/2, then with every turn the amount of reward the evaluation market gives to a move is cut in half. This goes to (almost) 0 quickly, so we can pay out the total amount of reward given to each move in only a few days.
Doing it continuously, the weighting function is just a smooth exponential rather than steps. We'd normalize that function to have an integral of 1 over the payout period. It will take a bit of calculus but given timestamps we can exactly calculate how much should be paid.