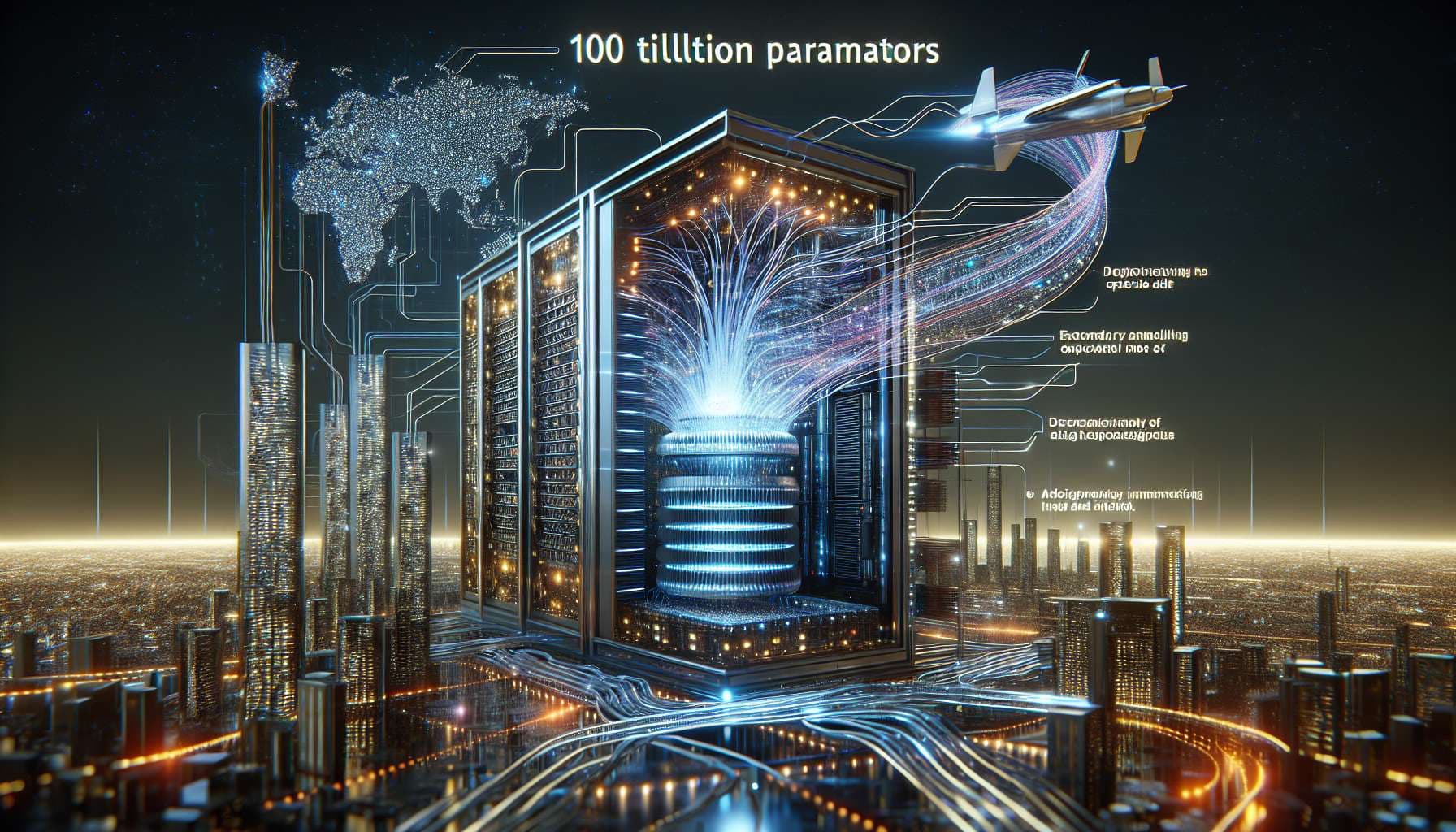
@Sss19971997 yes, if it reaches 100 trillion (I don't remember llama2 param count, and yes 1400 of them would be a shit ton)
@WieDan yes but b100s are presumably a lot more expensive too, and companies will take a fair bit of time to set up their clusters, especially if they recently set up h100s and then the model training for a 100tril param model is a lot of time too, don't think it'll happen
@firstuserhere GPT-1 was 117M, GPT2 was 1.5B, GPT3 was 175B (the trend with the old scaling law)
GPT4 was 1.8T with a MOE setup.
So historically param count has 10x'd per generation.
https://arxiv.org/pdf/2202.01169.pdf
I'm not looking closely at this paper rn and this predates Chinchilla maybe conceptually but it vaguely seems like performance boosts from experts saturate past GPT-4 levels although I'm not sure if this applies to inference cost/speed.
@firstuserhere You never said it had to be any good. Making a bad model with 100T parameters ought to be rather easy, as long as you have the space to store them (I do not, however)
@retr0id exactly. 100T might not be heaps, but it's enough to not bother with unless you think you're going to achieve something.
@firstuserhere The model exists before it's done training. It exists as soon as the parameters are initialized.
@Supermaxman Resolves to the best estimates possible. I'll take a poll of AI researchers at top 3/5 AI labs in that case.