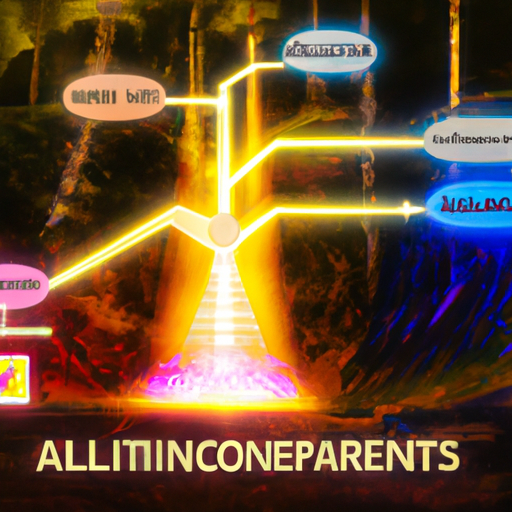
The launch of ChatGPT has demonstrated the immense potential of Large Language Models, making it impossible for business leaders to ignore the importance of ML/AI.
While product development conversations can be dynamic and fluid, at the end of the day, developers must create code that works, compiling into results that can be displayed on screens, which means actual software .
To help facilitate planning and conversations about what is needed at what time, please participate in this market.
Market Description
Which Part of a Full Stack Machine Learning Workflow Will Become The Most Breakout In 2023, measured by Github Star growth in a particular repo?
This page gives a good breakdown of various systems within an AI or ML productionalization architecture. We could grab repo's from here and track and bet on those: https://github.com/EthicalML/awesome-production-machine-learning#quick-links-to-sections-in-this-page
People are also trading
Using this tool: https://seladb.github.io/StarTrack-js/#/ I analyzed feature store as well. FeatureForm appears to have purchased fraudulent stars...?
Doing an analysis of everything so far, taking out the ETL's we get: https://star-history.com/#argoproj/argo-workflows&kserve/kserve&bentoml/BentoML&iterative/dvc&allegroai/clearml&catalyst-team/catalyst&aimhubio/aim&determined-ai/determined&pycaret/pycaret&Date ... which, DVC and Argo seem to have the most momentum behind them, having started earlier. The nest top, Pycaret, actually appears to be a super lightweight competitor to MLFlow and Kubeflow, working as a wrapper around Python, so it might not be considered purely a, "training application," as it does have some functions which allow for saving to the cloud. However, it might be designed more for rapid prototyping rather than full production use, but that could also be why it's more popular. BentoML appears to be beating out KServe, and ClearML appears to be narrowly beating out AIM and both appear to be well ahead of Determined. Within model and data versioning, Catalyst appears more limited in scope than DVC which has effectively no competitors.
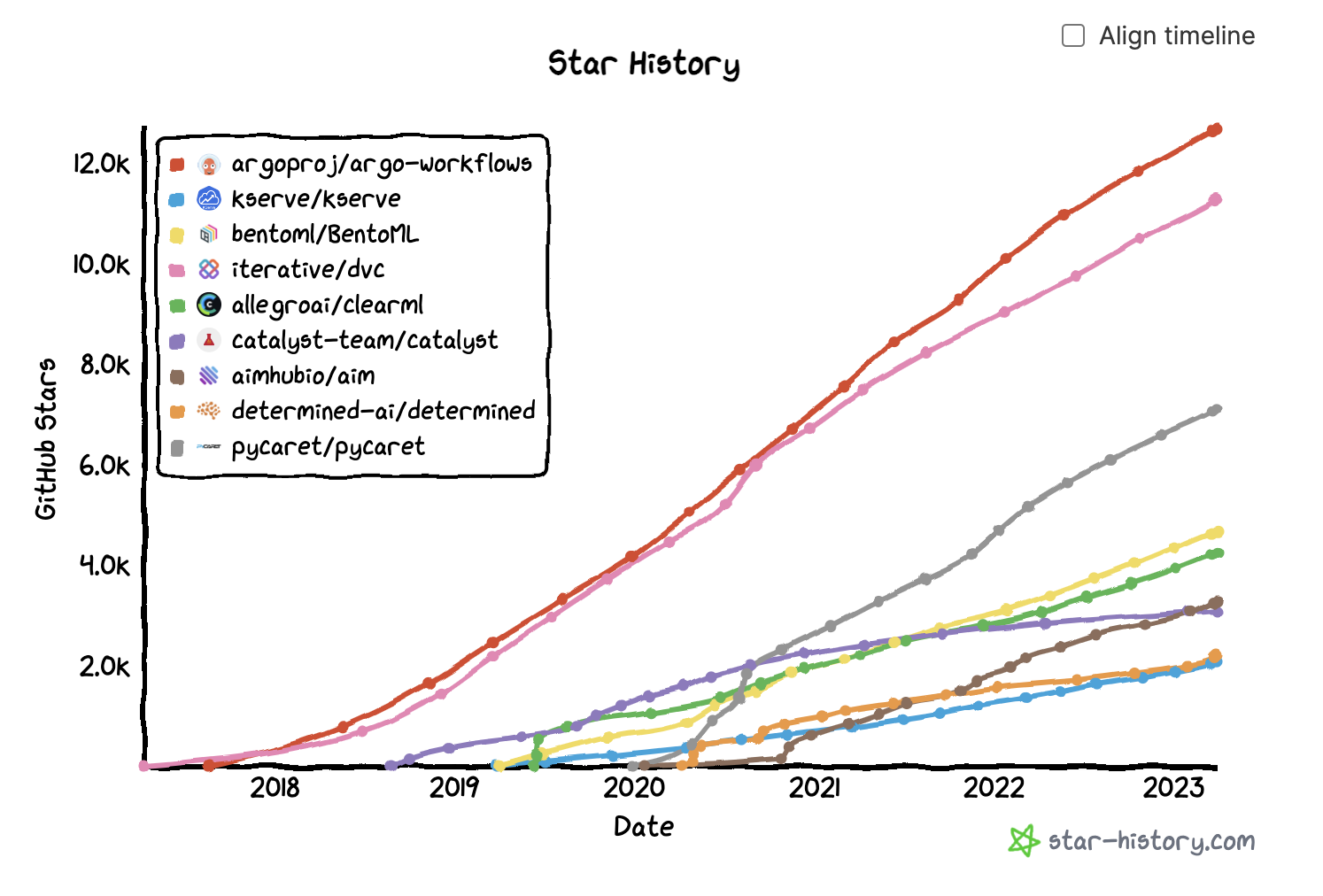
For this reason I'm putting most of my bets on Pycaret, which appears to be the most breakout tool at the moment ... prototyping, citizen data science.
Training Tracking & Eval Group: https://star-history.com/#aimhubio/aim&determined-ai/determined&pycaret/pycaret&Date
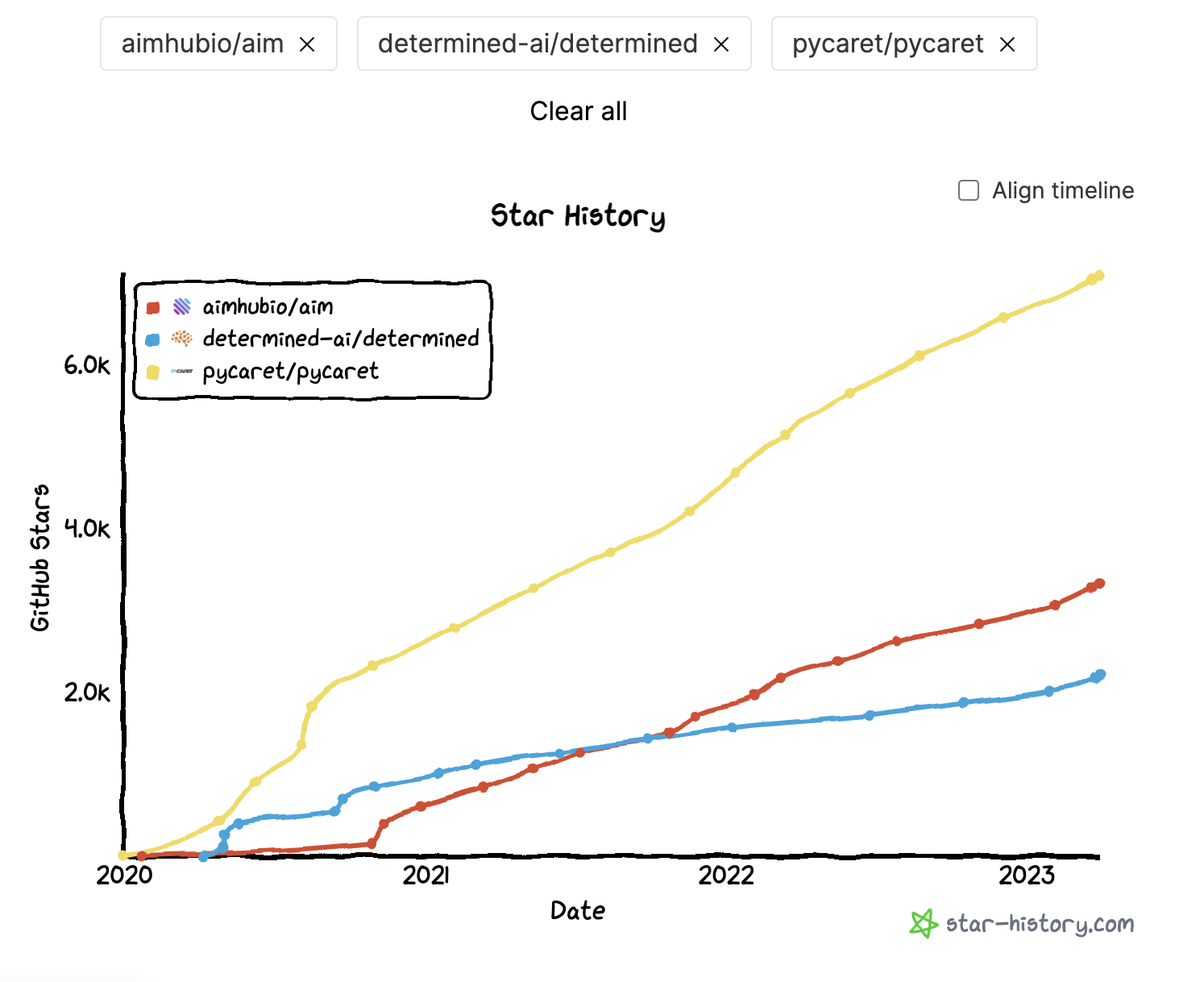
Model Versioning Group: https://star-history.com/#iterative/dvc&allegroai/clearml&catalyst-team/catalyst&Date
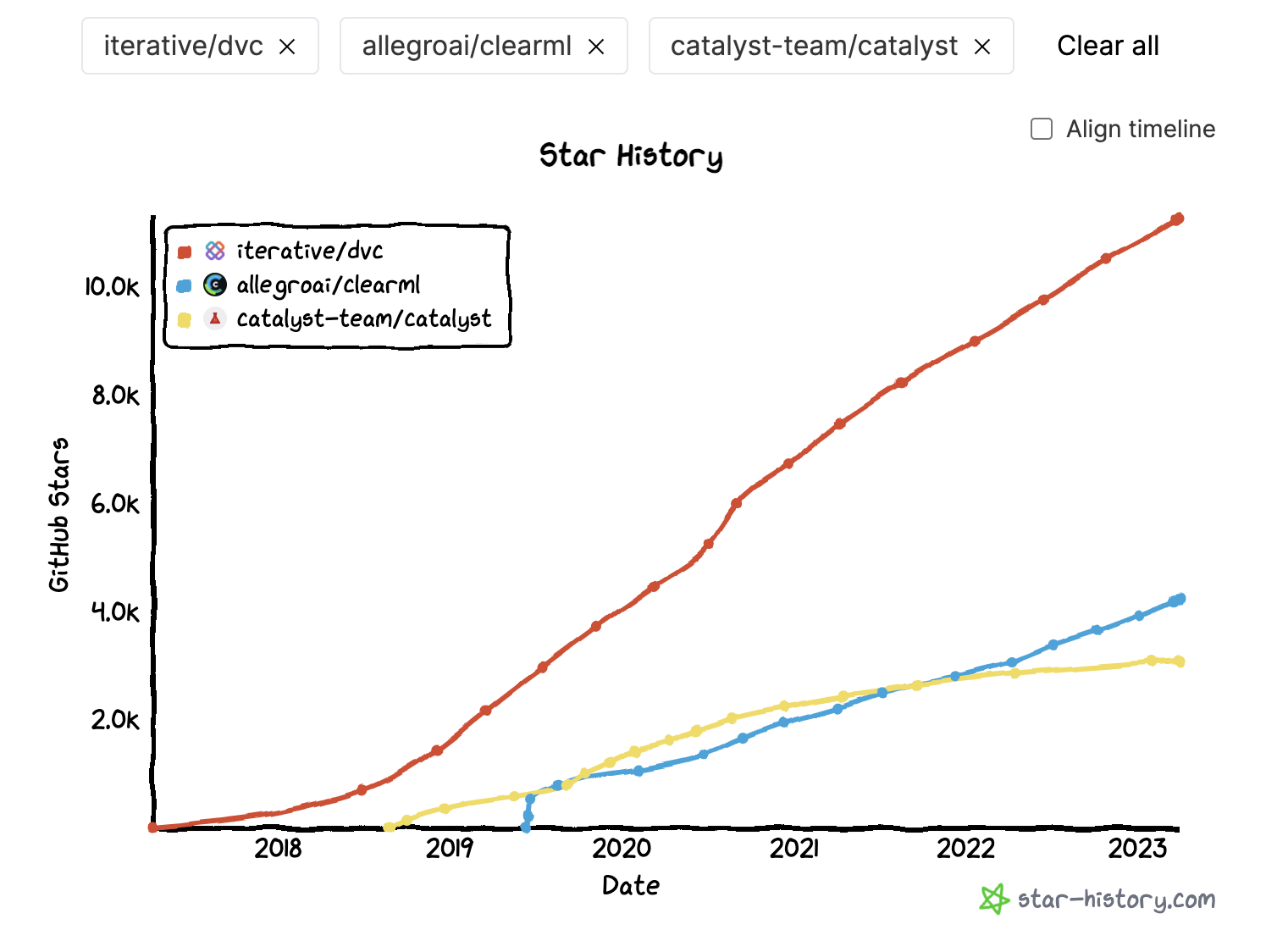
We can look at star history by looking at something like this: https://star-history.com/#feast-dev/feast&Date
This page gives a good breakdown of various systems within an AI or ML productionalization architecture. We could grab repo's from here and track and bet on those: https://github.com/EthicalML/awesome-production-machine-learning#quick-links-to-sections-in-this-page