
The LM must have 'frontier' performance (having PILE/or similar perplexity above one year prior's SotA). The LM must have been trained after 2022.
If it's unclear whether this has happened, I will give this a year to resolve. If it remains plausibly unclear the market will resolve N/A.
Fine-tuning includes all RL training. Training on synthetic data, or additional supervised learning (which is deliberately trained on after training on a PILE-like generic dataset) counts as fine-tuning. If the nature of pre-training changes such that all SotA models do RL/instruction training/etc. during the initial imitation learning phase, I will probably resolve this question as ambiguous. Multi-modal training of text+image will by default count as pre-training.
Related questions
For reference, current estimates of most expensive training run pre-2026 is at 10x GPT-4 price. https://www.metaculus.com/questions/17418/most-expensive-ai-training-run-by-year/
Meanwhile information on the data supply of text is available here https://epochai.org/trends#data-trends-section
Added some detail to clarify "Fine-tuning includes all RL training. Training on synthetic data, or additional supervised learning which is deliberately trained on separately from a PILE-like generic dataset counts as fine-tuning. If the nature of pre-training changes such that all SotA models do RL/instruction training/etc. during the initial imitation learning phase, I will probably resolve this question as ambiguous."
This does not really make sense to me given the purpose of pre-training in bulk knowledge learning, and fine-tuning in setting certain behaviours. In fact very little SFT data is required to get desired behaviour; certain papers such as LIMA have shown this.
Yann LeCun has a famous cake analogy that describes this.
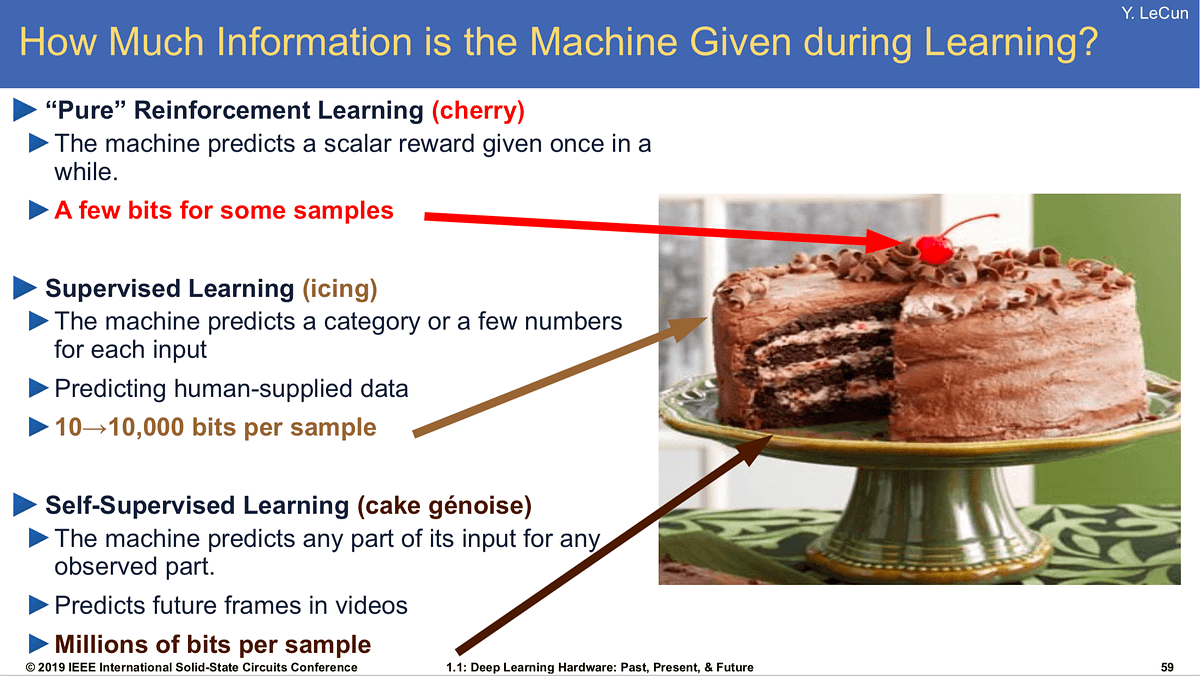
-