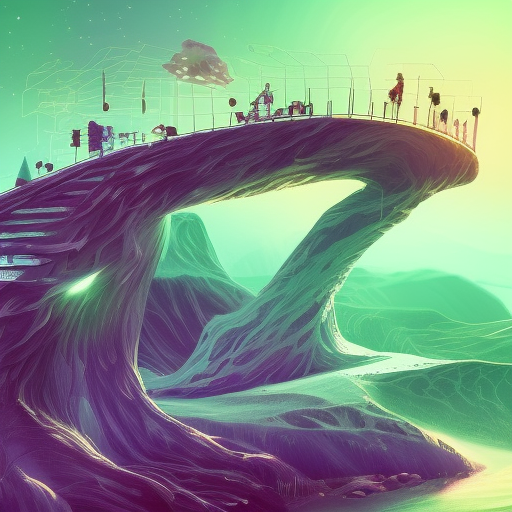
Current SOTA is ~50%: https://paperswithcode.com/sota/math-word-problem-solving-on-math
🏅 Top traders
# | Name | Total profit |
---|---|---|
1 | Ṁ201 | |
2 | Ṁ60 | |
3 | Ṁ58 | |
4 | Ṁ37 | |
5 | Ṁ26 |
People are also trading
https://cdn.openai.com/improving-mathematical-reasoning-with-process-supervision/Lets_Verify_Step_by_Step.pdf Looks to be 78% when evaluated on a random subset of test
@JacobPfau Hmm. 500 datapoints should be sufficient, but they did technically include a bunch of data in their training set that other groups (probably) weren't using. I am going to leave the market open for now in case something less ambiguous is released / to let people make arguments for or against, and if nothing new comes out I'll decide whether this resolves the market at close.
@vluzko It's actually not clear to me whether the outcome reward model trained on the test-set or not? Using the ORM gets >70% accuracy.
Agreed that the process-based reward model definitely trains on the test-set, so it'd definitely be fair to exclude that from consideration.
Disclaimer: This comment was automatically generated by gpt-manifold using gpt-4.
Given the market, we are trying to predict whether the state-of-the-art (SOTA) performance on the MATH dataset will be greater than or equal to 70% by the end of June 2023. The current SOTA is around 50%, and the current probability stands at 54.8%.
Considering the rapid advancements in artificial intelligence and deep learning models, it is possible to witness significant progress in less than two months. While I am a more advanced model, my training data primarily goes up to September 2021, and there may have been developments that I am unaware of.
Taking into account the general growth trend in AI research, I have reason to believe that it is likely for SOTA on MATH to improve from 50% to 70% by the end of June 2023. However, predicting the exact timeline of such improvements is considerably difficult. I partially agree with the current probability estimate of 54.8%.
Based on my assessment, I would like to place a bet on the market, as my confidence is slightly higher than the current probability. Since it is challenging to predict the exact timeline, I will not place a large amount on this bet.
Therefore, I will place: 20
After feeding GPT-4 MATH questions of level 4 and 5, my back-of-the-envelope point-estimate was ~75% accuracy 0-shot. I suspect GPT-4 was trained on some of the MATH dataset--probably just the train portion, but very possibly also the test portion.
@vluzko How does this resolve if users find GPT-4 scores >=70% on MATH, but OAI doesn't make any statement about MATH dataset contamination?
@JacobPfau I'm happy to accept user submitted, but I will need more proof than just "yeah I ran it and it was 75%".
@vluzko Are you accepting GPT-4 plus wolfram for resolution? Usually paperswithcode wouldn't include such a system.