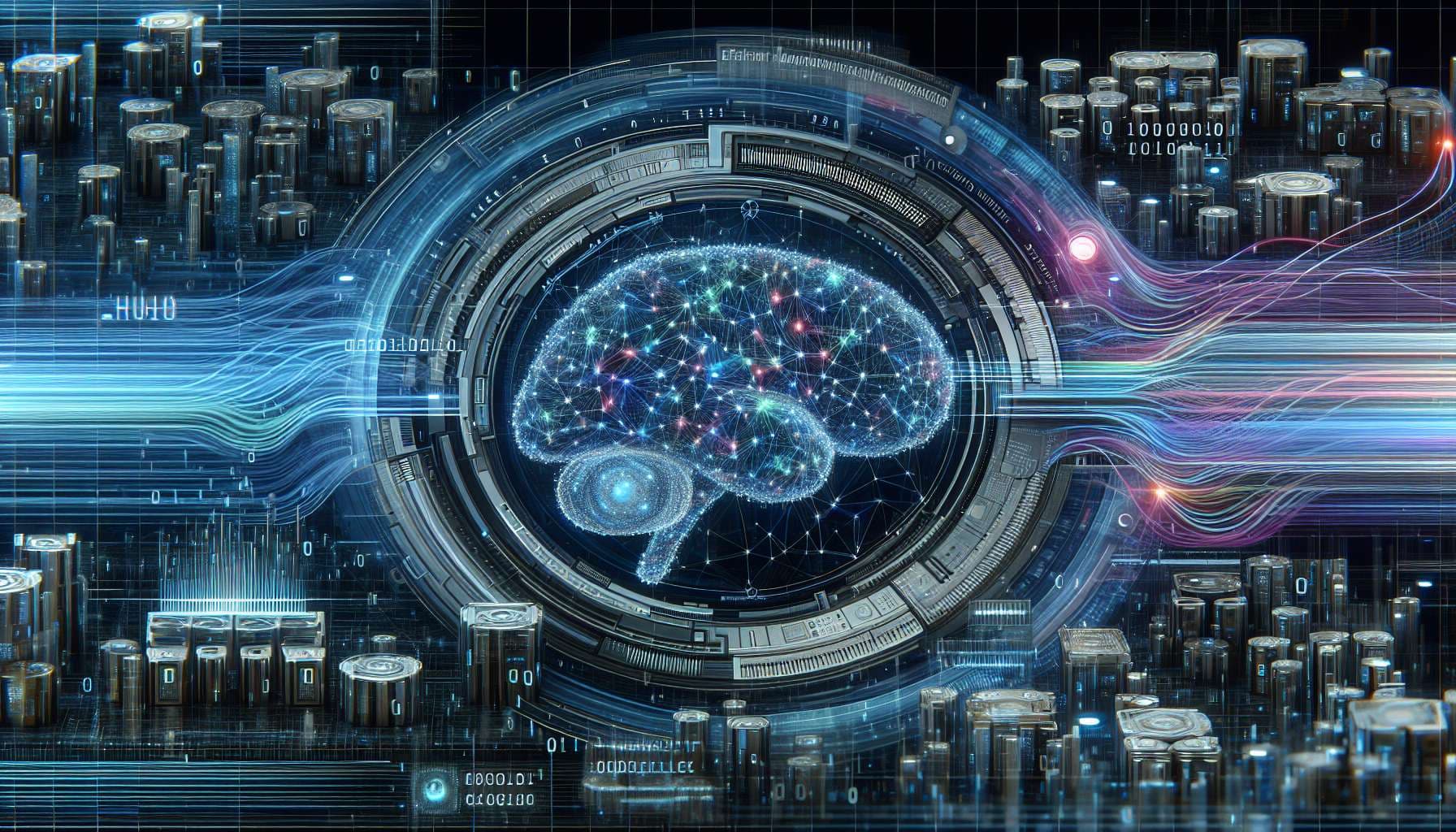
Resolves YES if deep learning models at the compute frontier* could be** trained using full homomorphic encryption (FHE) with a <10x slowdown before 2030/1/1.
[*] Say, within 1 OOM of the highest-compute model deployed.
[**] No need for frontier models to be trained with FHE. Empirical evidence of smaller models trained with FHE at <10x slowdown plus a heuristic argument (e.g. dumb extrapolation) that larger models would also satisfy this will suffice.
People are also trading
i could do 20k on no at 50 here but thats a big enough % of my portfolio i wont leave that up
@jacksonpolack likewise, strong NO from me, but I'm too poor to bet big bucks long-term
.
https://arxiv.org/pdf/2202.02960.pdf "HElib is [...] almost 19M times slower for multiplication". Like, these are state of the art results. I have no doubt that they'll continue to improve over time, but 19,000,000 is a loooooong way from 10