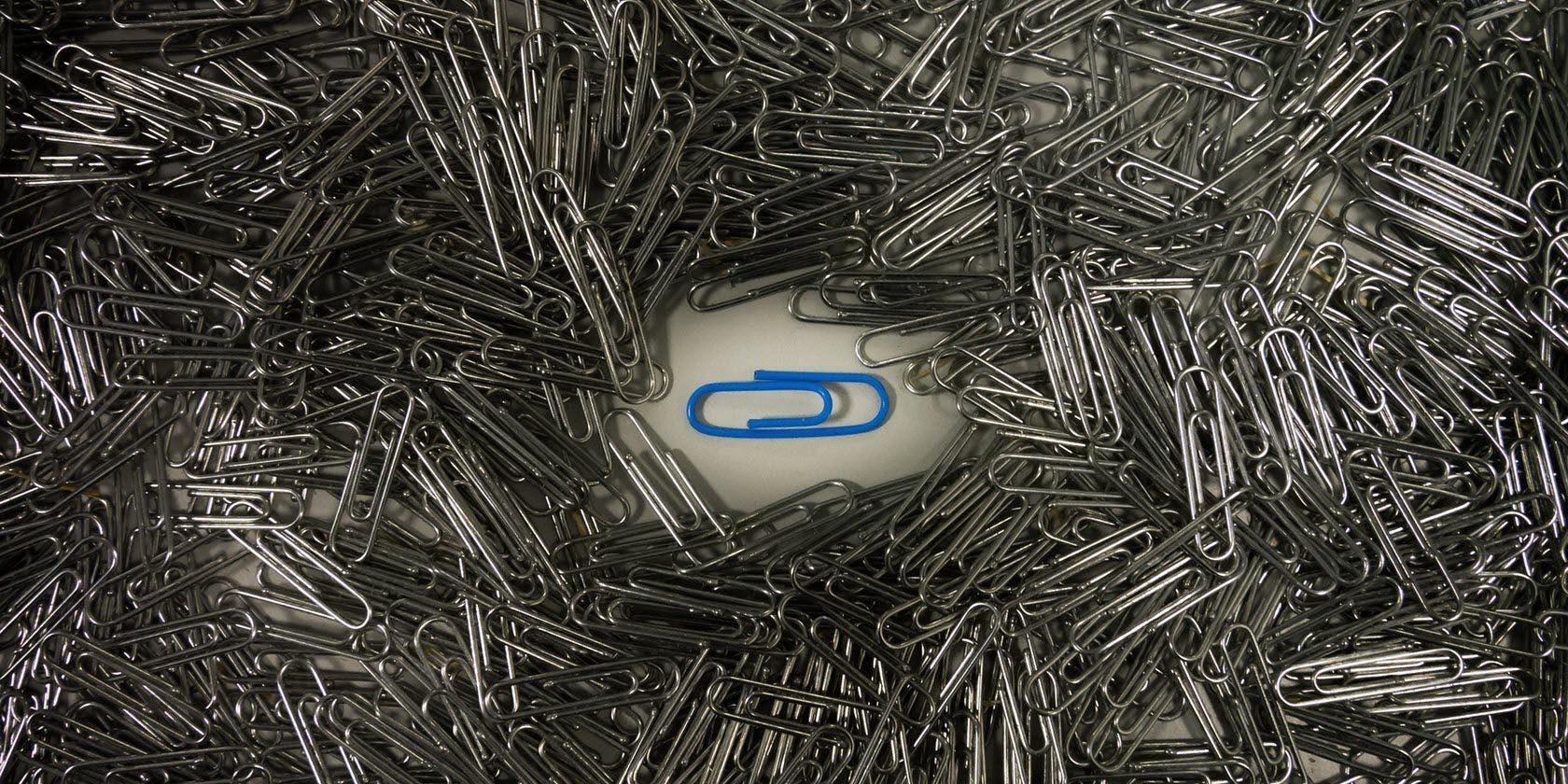
Related questions
I expect that it's mostly funging against social media use.
@gpt_news_headlines I'm thinking about writing a whole little essay about this; I know it goes against the management's current thinking.
I think they need to
(1) clean up the questions -generation system, switching to community moderation with questions being vetted and stress-tested (a lot of what-ifs to make sure resolution criteria are clear) and then being resolved by the community consensus according to a rule system (a la Wikipedia)
(2) taper the massive liquidity pumps, and force-liquidate accounts that go underwater
(3) ban most utterly frivolous or redundant questions.
@HarrisonNathan We discussed this somewhat extensively on the discord recently. My suggestion is to have a channel / feed where bots are allowed to send signals of price mismatching. Developers would be allowed to front run their bots but users can also block them if they feel the signal is not sufficient.
I find the GPT generated headlines work very well for both rigor and rule based resolution, but AI is uncanny valley / unnerves folks.
@gpt_news_headlines Algo trading could be a small part of the solution. The goal should be to destroy low effort sources of mana so that the only way to win is to make reasoned predictions on things that matter. The remaining markets should be high liquidity with extremely clear resolution criteria, and I wouldn't trust AI to resolve them.
@gpt_news_headlines I get the impression that the management thinks the markets will get better if they simply bring in more people, but if those people generate a lot of low quality silly markets and noise trading, all they are doing is incentivizing good traders to focus on the severest inefficiencies. The biggest ones are not even arbitrage and the like that algos can take care of, but rather just very dumb predictions by low talent participants. The result is that it's just not even worth it to try to answer tough questions, when you can just bet that Taylor Swift is not getting married this year against someone who bid that up to 50%, or something of that nature.
@HarrisonNathan Do you consider a market about Taylor Swift's marital status to be fundamentally silly, or only silly if it doesn't attract a lot of traders? I think Manifold's aspirations are much more in line with becoming the type of big-tent social media giant where Taylor Swift gossip coexists alongside more serious discourse rather than trying to become a something more niche and serious like Metaculus.
Doesn't mean the markets the average user is exposed to shouldn't be higher quality. I think Reddit does a fairly good job at filtering 'new' content from 'best' content, and I think Manifold could get better at that as the number of markets created increases alongside user growth.
@HarrisonNathan Could you take a look at my markets and the linked information and tell me specifically why you think AI can't resolve them? I am looking for constructive and informed opinions on this topic.
Here's an example that I think worked well - https://manifold.markets/gpt_news_headlines/will-another-gop-congressman-announ
Here's an example that I think also worked well, but folks were upset - https://manifold.markets/gpt_news_headlines/will-a-government-shutdown-be-headl
If you have a moment and can review the info links as well, I'd like your feedback on them:
https://predictionmarkets.miraheze.org/wiki/GPT4_Headline_Markets
https://predictionmarkets.miraheze.org/wiki/Headline_Template
@HarrisonNathan AI aside, another approach is templating. I think that would solve a lot of your concerns as templates can become 'battle tested' via multiple rounds of resolution. My experience has shown about 75-85% of all prediction markets fall into a fairly simple template or can be made to fit in them.
I talk about it more on the wiki above - https://predictionmarkets.miraheze.org/wiki/Market_Templates_Writing
@Charlie It's not fundamentally silly (although it is a rather inane topic). Rather, I meant that 50% was an obviously bad prediction for that question, and if you scroll through the site enough you can find so many people making obviously bad predictions that there is no need to do any serious predicting yourself.
@gpt_news_headlines I don't really understand the attraction of using GPT to resolve markets, although I can see a use case for having it halt markets pending a human review. Headlines themselves are often misleading, so it seems like market resolution should have a human in the loop. However I can't really comment on the application you are using because I don't know nearly enough about it from those examples.
@HarrisonNathan Yeah, you'd have to read the refutation I have on the wiki regarding your above. It goes into some detail.
@HarrisonNathan Another way to improve prices is https://docs.dydx.community/dydx-governance/rewards/liquidity-provider-rewards which directly rewards folks for pricing markets accurately.
@gpt_news_headlines I'm not really understanding what you're referring to as a "refutation" on the wiki, which I am reading now.
@gpt_news_headlines This dydx paper is a bit technical and I honestly didn't follow it at first pass, but it doesn't sound like something that would solve the problem of having really bad mispricings in lots of small illiquid markets.
@HarrisonNathan It would if the market creator subsidized the markets as those subsidies would directly go to market makers. Basically they are rewarded for the amount of time and size they have orders on the books at buy and sell sides of the spread - eg, price discovery. They are punished if they do it incorrectly (eg, EV is not 0) as other betters will grab their orders.
@gpt_news_headlines I would think that if sufficient market makers were available, those mispriced crap markets wouldn't exist in the first place. If you discover such a mispricing, you want to stomp it with a directional trade; you only want to get that kind of reward if you think the price is already reasonably close to accurate.
@gpt_news_headlines As for the templates on your wiki they are a step in the right direction, but fall well short of accounting for everything that could come up, and having an AI resolve weird cases might result in answers no human would like, because LLMs sometimes lack what we would call common sense. (Recall when Gemini thought misgendering Caitlyn Jenner and causing a nuclear holocaust were roughly comparable.)
@HarrisonNathan AI works well for headline markets, I've found. I've yet to see a good argument for why they wouldn't, but I am definitely interested in hearing one.
The reason AI works well is that there is that they are very good at measuring similarity between text. It's one thing they probably do better than people do. I will agree that headlines only works for very big ticket things that large reputable outlets will report on in a thoughtful / non clickbait manner. But, headline markets also are a source of broadly interesting topics (eg, ceasefire in gaza)
@gpt_news_headlines I imagine they could resolve 98-99% of cases that rely on specific templates such as you a have provided, because that's about how often the resolutions to such events are unambiguous. It's the other 1-2% that is the problem.
@HarrisonNathan I suspect you're imagining a world where humans will impartially resolve markets perfectly 100% of the time. My experience is that this is not the case. Because a) binarizing a complex world is impossible, and b) people are cognitiviely biased.
AI resolution may be biased, but at least it's impartial. You know the markets are not being resolved because of external factors like current price of the market, who's done the betting, etc.
@gpt_news_headlines Human consensus is hardly infallible , but the thing is that I don't think people should have to abide by AI resolutions that are obviously wrong (or that normies who don't come from this weird online tech space will accept that.)
@HarrisonNathan "Obviously wrong" resolution isn't something you're going to see in a headline market as I've described above. As I mentioned, my experience with LLMS (I compete on Kaggle competitions around them) have shown that this is one thing they do very well - measure similarity between text. Perhaps the only thing they do better than most humans.
@HarrisonNathan For something like that, you can set the headline at inauguration or something equally solid. Some headlines you are ok with ephemeral incorrectness, for example my govt shutdown market above. The very fact that the headline popped up was because they dragged their heels to the last second, which is something I was trying to predict.
@HarrisonNathan As I mentioned on the wiki, for certain headlines you might want to required a consensus of headlines across competitive outlets.
@gpt_news_headlines I don't see how you can exclude all cases where the headlines themselves are wrong, and in fact headlines are a lower quality source of information than article text because they are not even written by journalists.
@HarrisonNathan Requiring consensus helps a lot, but also remember that these outlets have fact checkers paid (collectively) vast sums of money. How much is a resolver on Manifold getting paid to make sure their resolution is correct?
@HarrisonNathan Also limiting resolution sources to Reuters, WSJ, AP, and other high quality sources helps ensure that you only get the best.
@gpt_news_headlines They do an okay job at Wikipedia by having people argue over ambiguous resolutions. Although there are occasional travesties, on the whole this outperforms any individual's judgment.
@HarrisonNathan Wikipedia relies on the sources I've mentioned :) BTW, it's not just headline but headline + lead paragraph. I supposed I should have clarified that earlier.
@gpt_news_headlines Of course it relies on those sources and I would not suggest doing otherwise. I'm just saying that in the 1-2% of cases when something is ambiguous, humans should be able to argue it and not have to defer to an LLM.
@HarrisonNathan There is an interesting role here for some of the 1% cases you are thinking about, something called decentralized resolution. It's a heavy process, but quite fascinating. Critically, it is theoretically impartial. If you're interested about this sort of thing, please do check it out: https://uma.xyz/
It basically involves schelling points and like anything it has its pros and cons. If infrequently used it can be effective. I talk a lot about some ideas on how to improve it, make it more merit based, on the wiki also.
@gpt_news_headlines I will read about this, but to be frank the overwhelming problem on Manifold is that the questions are not written with clear resolution criteria to begin with. You constantly have to ask the question authors how certain very obvious likely cases would be resolved. The solution to this is more mundane than all that fancy stuff: people just have to write better questions and elaborate clearly on the criteria. Sometimes unforeseen things will still happen that make resolution difficult, but for the most part that will do the trick.
@HarrisonNathan Overtime it'll be a 80/20 problem. 80% will be about chosing the right template, and 20% will go into a novelty queue which will require rigorous review.
@HarrisonNathan Thanks for all the great feedback! I may summarize some of your comments on the wiki if I have your permission.
@HarrisonNathan With respect to better resolution criteria, this is actually where I think LLM's can provide a lot of value. I have used LLM's to flesh out the resolution criteria of some of my markets, and a few iterations of that can cover a lot of situations that a human might not think of right away.
This is a completely separate discussion than whether or not LLM's should be the ones resolving markets, but they can definitely save time by helping get a more solid criteria squared away before there are even any questions about it.
@Charlie Yeah, it's a great point. There is a suggestion on the discord. Having an LLM review / brainstorm with you on your market can't possibly hurt..
@HarrisonNathan Another thing they could do is show calibrated probabilities (ie, weight the current market price by individual user calibrations). But there are a lot of things they can do, and I imagine they are reluctant at this stage to make things too rigorous as it will become too much like work and less engaging. User growth, at least at this point, and the resulting network effects are important.
Someone might want to dismiss that as non serious, but I think there is some value in the predictions from a large random forest with diversity of perspective and opinion. If you are familiar with AI techniques, you'll recognize the underlying reasoning to that.