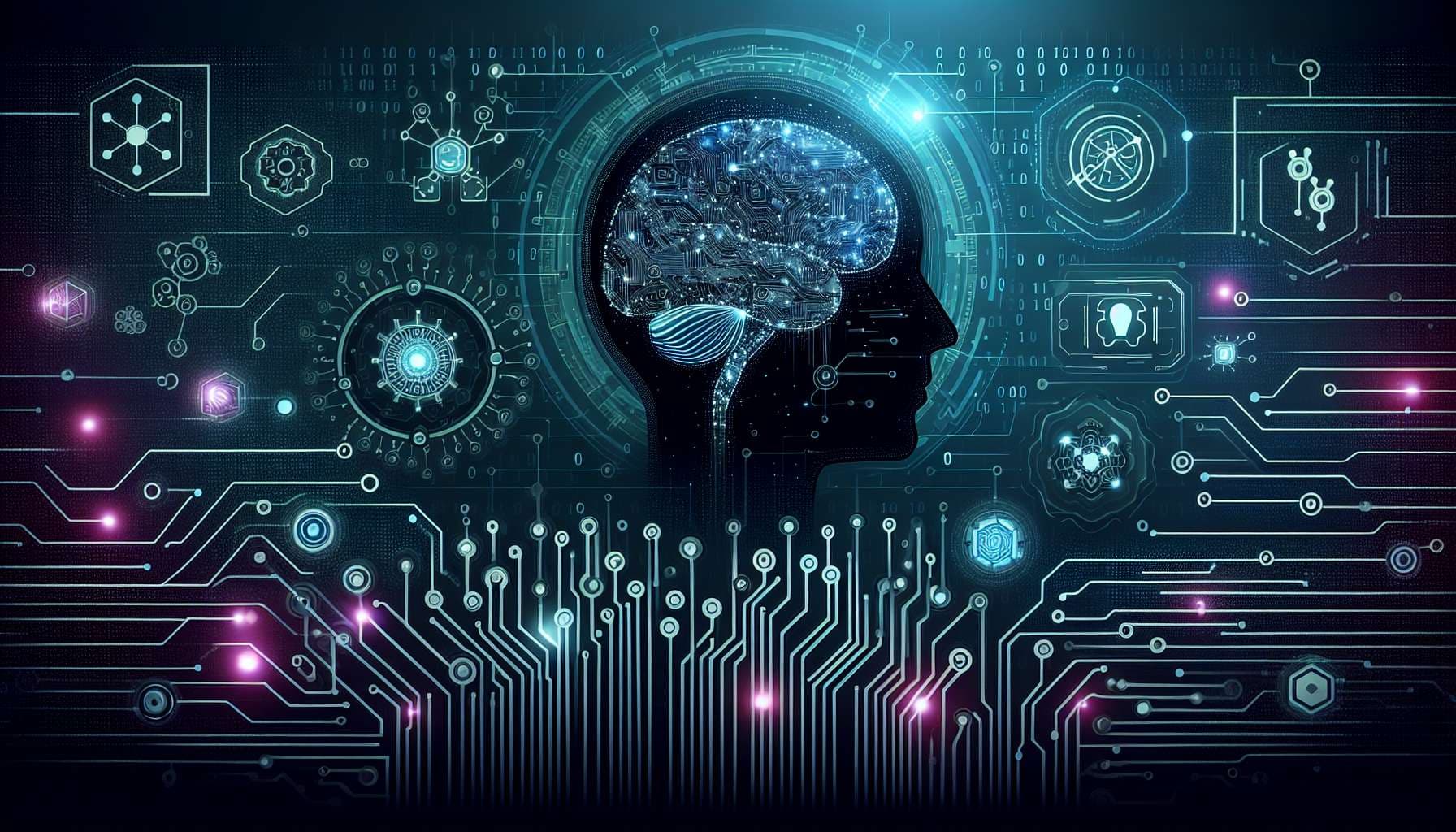
This is a market about whether a preserved human brain will be digitized (at the resolution of synapses, and with a fidelity estimated at >90% of synapses). This is not for doing anything with the data, just getting it onto a computer.
To qualify, the dataset would need to include: the whole connectome (e.g. neurons reconstructed from scanned slices), approximate synapse strength (this comes through in electron microscopy, and certain molecular labeling regimes), the cell type (e.g. Glutamate vs GABA vs dopamine, etc), and the degree of myelination of axons.
I believe that this would constitute sufficient information for an accurate emulation of the scanned brain, but determining whether that is true isn't part of this question.
Related questions
For those interested in more details about what has been done so far, check out this video: https://youtu.be/vTyqKHpueLs?si=4eY8F_0hbcmNrPuH
It is indeed a massive undertaking. Without AI there would be no hope of accomplishing this task in 100 years, much less in just 11.
A brief video to provide an intuition pump of where we are at today: https://youtu.be/blaS5fBJdsE?si=HjcdSV1Qht2k6pVG
@NathanHelmBurger so human brain is 1,300,000 cubic millimeters. You need several more generations of robotic equipment to make this faster to scan and then billions in funding and a lot of time to hand build the facility that does it. As I mentioned below, the facility is similar to an IC fab but a new design, and the end product isn't profitable.
It's going to happen someday but unlikely in 11 years unless the funding (10-100 billion?) Is already allocated.
What you could do is sample the brain this way, develop a complete model from lower resolution micrographs of the areas not scanned in detail, and essentially steal the brains design. This won't have the memories or personality of the original individual but should have the same general intelligence.
@GeraldMonroe Yes, using the brain imaging technology of a decade ago, you are correct. You are making assumptions about the brain imaging technology itself not advancing. I have reason to think your assumptions in that regard are incorrect.
What if it were possible to scan, in detail, a 1cm thick slice? What if it could be done using a new type of optical microscopy rather than electron microscopy? What if the field of imaging for the width of the slice were around 1cm^2. What if the 1cm thick slice could be left intact with its neighboring slices such that you only needed a gantry to slide the slice past the microscope? What if the tissue had been stabilized in such a way that it was much more resistant to physical damage or distortion?
If such assumptions were true, then the costs would suddenly seem much smaller. A few million dollars perhaps.
@NathanHelmBurger so 1 cubic centimeter and in the viewing window there are 66.2 million synapses on average. The critical regions are likely much denser. You mean to extract the strength of each connection, and you have to know the type.
There are also approximately 40 neurotransmitter types, and each has around 9-20 known receptor types. So there's thought to be hundreds of permutations. You need to stain them all, so imagine all these synapses glowing slightly with laser light onto your sensor outside the cubic volume, where you visualize each with a different frequency of stain. (But there will be interference...)
I am not an expert here just noting you are going to be up against wavelength of visible light limits and many many valid interpretations from your data. Would need more data to know if it's feasible. Possibly not at the volume size you have chosen.
Later in the Singularity if robots can build each other sure, just use a square kilometer facility and scan a patient every day or so. I am not disputing it couldn't be done but you need exponential amounts of scale.
@GeraldMonroe Good thoughts again! Also things that are in my model. Lets say that this stabilized tissue sample could be stained, have the stain washed out, and stained again, without damaging the tissue. Thus, using three distinct wavelengths of light, you can acquire the 3D positions of two new proteins registered against 1 previously scanned reference protein. Repeat for all proteins of interest. Laborious, yes, considering you'd have to do this for many proteins, and repeat the whole process for a couple thousand rectangular prisms of tissue. A big project. But, I think, solidly in the < $100 million range.
@GeraldMonroe You've been so helpful in setting up objections for me to respond to, it's very satisfying. After finishing my comment responding to you I found myself waiting eagerly for the next most obvious objection to be raised. Next Objection: What I am discussing seems to be using optical microscopy to resolve individual synapses. This is not physically feasible because synapses are (slightly) below the minimum size for optical magnification, the 'diffraction barrier'. If you can't measure synapse size, then you can't measure synapse strength, which is a key part of the necessary information. (maybe @Amaryllis wants to know?)
@NathanHelmBurger Fine, fine, nobody wants to play. I'll answer my own question then: https://en.wikipedia.org/wiki/Expansion_microscopy
@NathanHelmBurger I am not sure you can do this at all. Just keeping then sample "liquid" enough to wash is damaging it. The proposals I read were very thin slices and you may stain at most once.
I knew there were methods to extend the range optical microscopes can cover but at this point you aren't directly getting image data but Indirect information on the path the photons took. This probably does not work at all on a thick slice.
@GeraldMonroe Not sure which papers you've read on it, but here's a recent overview of some of the current leading techniques:
https://www.ncbi.nlm.nih.gov/pmc/articles/PMC8121164/
The last sentence of the paper summarizes it nicely:
"Finally, clearing, labelling and imaging of adult human tissues on the order of centimetres will be one of the primary technologies to reveal the cellular structure of whole organs and eventually map circuits in whole human brains."
And here's a paper detailing immuno-staining in clarified human brain tissue: https://bmcbiol.biomedcentral.com/articles/10.1186/s12915-023-01582-6
So 11 years and the largest organism scanned is under 1000 neurons?
Even an AI Singularity doesn't necessarily make this happen. Suppose there are prototype AGI in 2026, robotics is mostly solved in 2030, and robots begin to build other robots though parts of the production chain are not automated.
Then 2 doublings from the starter number of robots plus human effort gets you a few hundred million robots.
You are going to need many beam electron microscopes (I recall the paper study on this was 1000 microscopes with 1000 beams each) and a large facility of the equipment that hasn't been developed yet. I think several thousand tape collecting ultra microtomes, and the facility has to keep the samples cold and it's also a clean room- it's similar to a chip fab but all custom. The sliced brain tissue is being stained and loaded for scanning into the microscopes.
All this, and the scan itself will still take several years per specimen.
The few hundred million robots are likely all at work doing less difficult tasks, human technicians have to build the above and there's not billions of funding allocated yet.
Chance is low, under 5 percent.
@GeraldMonroe Good thoughts Gerald! I often appreciate your comments. I think, given what you know, that you are right to be skeptical. However, insider trading is allowed on Manifold, and I may have insights into neuroscience technology that is not yet well known. What if, for instance, it turned out that electron microscopy wasn't required? What if even thin slicing wasn't required?
@NathanHelmBurger you mentioned on lesswrong this is singularity dependent, however. And there are physical laws involved and limits in reconstruction resolution that are properties of the equipment used, a smarter algorithm can't do better than theoretical limits. This is also a domain at the bleeding edge of what humans can do at all, it's going to be among the last things agi or robotics are able to contribute to.
Another factor, amusingly, is if an AI Singularity happens all the research funding and attention will probably be on that. It could suck away the funding needed to develop whole brain scale scanning on top of whichever volumetric method you are thinking of.
@GeraldMonroe Not singularity-required, just hard to imagine it NOT happening if the singularity did happen. I personally think this is still pretty likely to be an accurate prediction even in an AI-fizzle world.
@NathanHelmBurger I think it's really important to have a grounded model for what the Singularity can do. The one I constructed assumes 80 H100s per "person equivalent" and this let's you model how rapidly new "workers" added and new robots added. And robots (and the entire supporting industrial infrastructure) can double every 2.5 years.
You can construct a variety of models depending on assumptions but the main takeaways are your assumptions should be based on real data and plausible extrapolations.
And if you do this, the Singularity only makes a profound difference later, you need enough doublings that the robot population is effectively adding another China every few years...or every few weeks later on. And with 2.5 years per doubling that can be 2050s.
@GeraldMonroe I spent several months in 2022 meticulously crafting my own model of future compute necessary for a 'person equivalent', and the tech costs thereof. I'm not going to go into details here, but I will claim to have thought carefully about it and taken notes from reading a bunch of academic papers across multiple disciplines.
But the thing that haunts me about thinking about future 'person equivalent models' is, I don't have a good model of what happens if you scale up the amount of compute you are putting into a single model. Let's accept your model for a moment, 80 H100s. Like, if you figure out how to make a really good mixture of experts that can tolerate intra-datacenter network latency, do you then have the ability to run a model on 1000s of H100s and get something vastly more intelligent than the smartest human? I'm pretty sure it won't be that simple, but also, I'm not at all sure the many researcher-years that the individual 'person equivalent' models devote to the problem won't be able to overcome the obstacles for training and running an 8000 H100 model.
@NathanHelmBurger well it's diminishing returns. 100x compute if we assume the scaling is similar to gpt-4 at the edge of the curve, gives you approximately 2.67 times lower error rate. Whether or not that is worth 100 times the inference cost depends on the cost per error. (Heart surgery yes, assembling iPhones maybe not)
Note thats simply assuming the relationship is logarithmic and the log base is per this one example. A better model can be constructed with more data.
Yes, another factor is 100 times compute means about 20 times boost in serial speed, up to a limit determined by hardware latencies. You can also reduce the hardware latencies a lot and not throw away compute potential using custom hardware like groq.
More serial speed means faster discovery of a close to optimum algorithm capable of running on your hardware, yes. Though not by 20 times if you model the discovery process.
For example suppose AI researchers spend 1 month in serial time on a training run, and then 1 month updating their approach based on the results. Then with 20 times speedup using AI to research itself it's 30 days days per training run and less than 1 days to modify for the next shot. (Not 1.5 days because AI doesn't need sleep)
So slightly less than twice as fast, not 20 times faster.
There are some other benefits AI will have researching itself such as vastly larger working memory and of course it benefits from any cognitive improvements to itself...
Main takeaway is you have to be methodical with your models, use actual numbers, be able to justify them all from existing data, and don't reason on vibes or hope.
@GeraldMonroe Agreed on reasoning from actual numbers. But there is one point in the model you made that doesn't hold. "If we assume the scaling is similar to gpt-4" doesn't apply to my concern. My concern is explicitly that if a human mind were scaled up 100x (without adding problematic latency) I would expect my higher quality thoughts to emerge, something qualitatively beyond 'lower loss on next token prediction'. The concern I am phrasing is whether a somewhat more brain-like future AI algorithm (not just a bigger transformer) would experience gains from parallel compute more like this imagined 100x human brain.
I agree that we don't have enough information at this point to be confident that this will be the case. However, I also don't think we can rule it out.