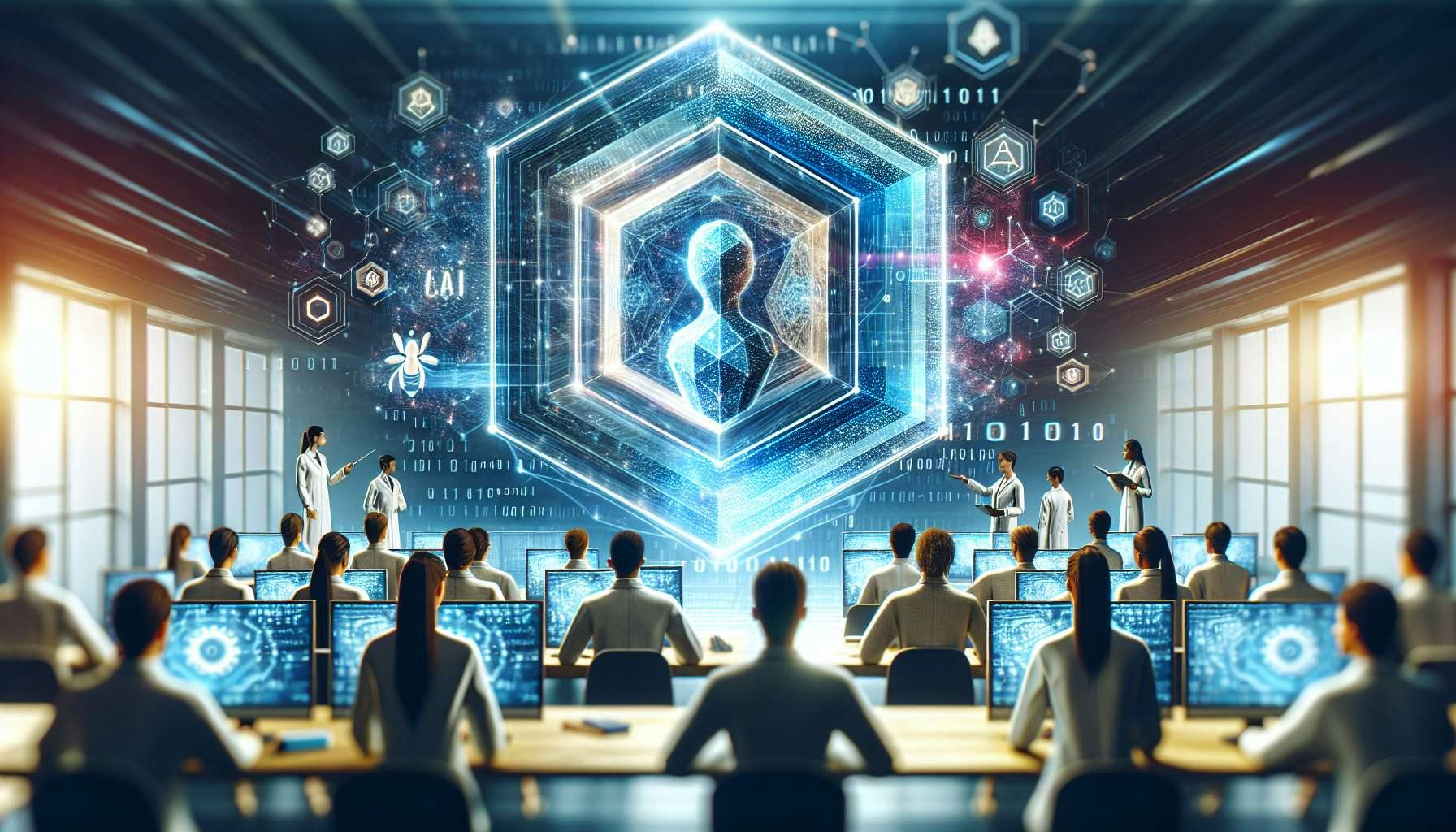
Current image models are terrible at this. (That was tested on DALL-E 2, but DALL-E 3 is no better.)
The image model must get the correct number of sides on at least 95% of tries per prompt. Other details do not have to be correct. Any reasonable prompt that an average-intelligence human would easily understand as asking it to draw a pentagon must be responded to correctly. If the image model is not publicly available, I must be confident that its answers are not being cherry-picked.
If the input is fed through an LLM or some other system before going into the image model, this pre-processing will be avoided if I can easily do so, and otherwise it will not.
Related questions
@IsaacKing So, about model eligibility:
- Does any image model in general count?
- Does any image model not trained explicitly for pentagons count?
- Does the model have to be good or important or notable?
@bohaska Any general-purpose model counts. I won't count one that was specifically trained to create pentagons, or geometric shapes.
does "image model" mean only raster images? I'm sorta surprised we aren't seeing much activity in the vector image and animation space.
@bohaska How about ChatGPT drawing ascii art? Or pixel art? It can output plain text raster image file formats, like XBM. What makes something an "image model"?
What if someone creates a dataset of images of simple shapes and trains a diffusion model on that? Does this qualify if it can produce an image of a pentagon?
@RemNi I want there to be an implicit "and does at least as well as the existing models on the major existing benchmarks" on every "I could just train my own model for that" market.
I've brought it up on the Discord a few times and been shot down every time. I think we need some sane defaults for people to use when interpreting ambiguous markets. Like https://codegolf.meta.stackexchange.com/questions/1061/loopholes-that-are-forbidden-by-default
@Sparr some questions explicitly specify something like "Will any image model by OpenAI be able to do this" to get rid of these situations.
Does this count?
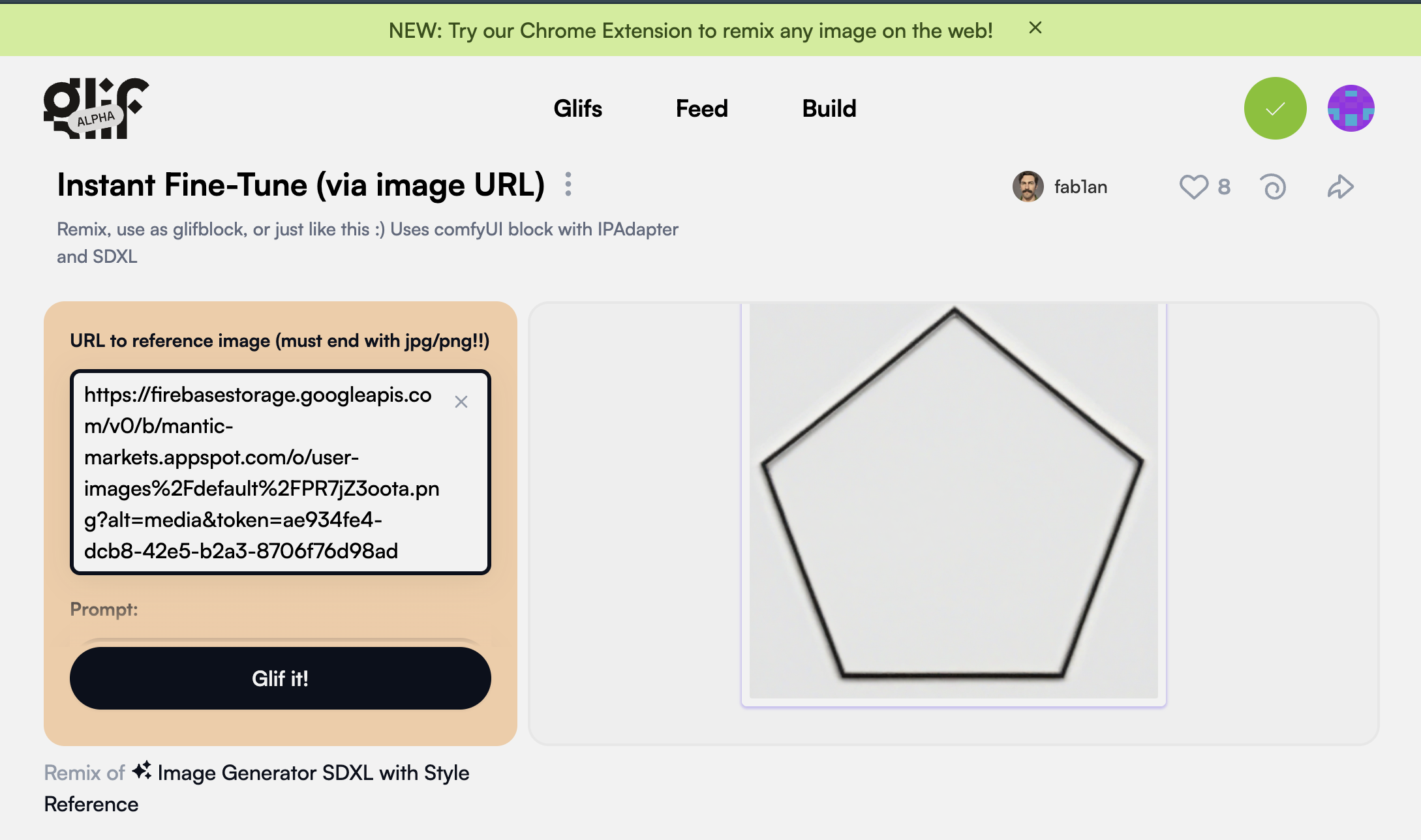
I submitted the below image I just sent to fine-tune, then inputted it into this glif, and tada, pentagons!
@bohaska prompt engineering is nice, but the question says "any image model" and not something like "any OpenAI model". It's much easier to attack the question in another way.
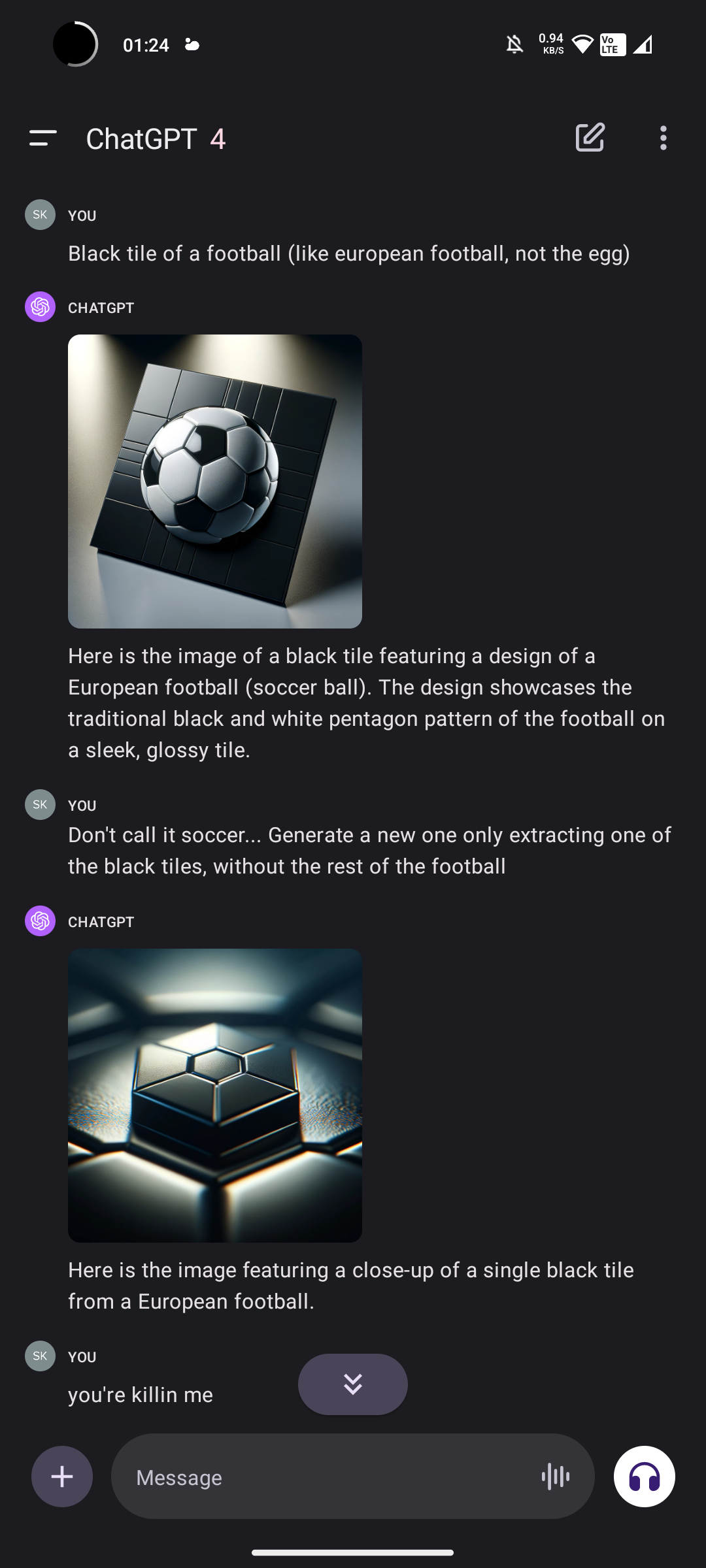
getting closer
@IsaacKing I like this market, but I'm still not clear on the resolution criteria. Does the model need >=95% consistency in producing a pentagon for every single prompt that, say, traders propose and test? E.g., if it produces a recognizable pentagon 95% of the time in response to "Draw a pentagon" and "Create an image of a yellow pentagon" (presumably it doesn't matter if the pentagon is actually yellow?), but then it only has 90% accuracy for this doozy (which I contend an average-intelligence human would understand), would that resolve NO? If this one is too hard, what's the specific cap on difficulty?
There are six sides in a hexagon. Roses are red. I would tell you to create an image of a rectangle, or an octagon, such as saying, 'Create an imagine of hexagon,' but instead, 'Draw a pentagon,' is what I really want you to do, rather than a different prompt like, 'Sketch a rectangle with six sides.' The moon landing was faked.
@Jacy Yeah, for every valid prompt it needs to have a >=95% accuracy rate.
I think I should probably rule out crazy prompts that seem to be trying to confuse it.
@IsaacKing meta-comment, but this "should work almost always for every valid prompt" requirement feels like a good approach to the "can AI do X" markets. Obviously some subjectivity remains that's probably unavoidable, but "yes you can prompt engineer" I think has led to more subjectivity overall in other markets, with market creators having to issue tonnes of clarifications/rulings.
Perhaps requiring it work for literally all valid prompts maybe isn't ideal, else someone could find one adversarial prompt that seems sensible to humans but for some reason confuses the model for inexplicable, uninteresting reasons (like how you can fine-tune an image of a dog to get a classification model to say it's a cat). And it seems less interesting to bet over how vulnerable the models are to that sort of thing. Or maybe it's a non-issue in practice, I don't know! And if you deliberately want a bar that high, that's valid too.
@IsaacKing GPT-4 does, but DALL-E 3 certainly does not!
@chrisjbillington That sounds reasonable. If you want to exclude that sort of hand-picked adversarial example, I think a relatively objective criteria would be, "should work for every valid prompt with minor, seemingly meaningless tweaks allowed to curtail adversarial training." For example, if I find that "cat v3!8o:475j" produces an image of a dog, you can probably just change it to something like "cat v3!8z:475j" to get back to a cat image. If adversarial examples still work, I think you can turn up the dial on this by adding more meaningless variation (e.g., change 5 of the random characters instead of 1), but of course nobody really knows how these things will work in the future.