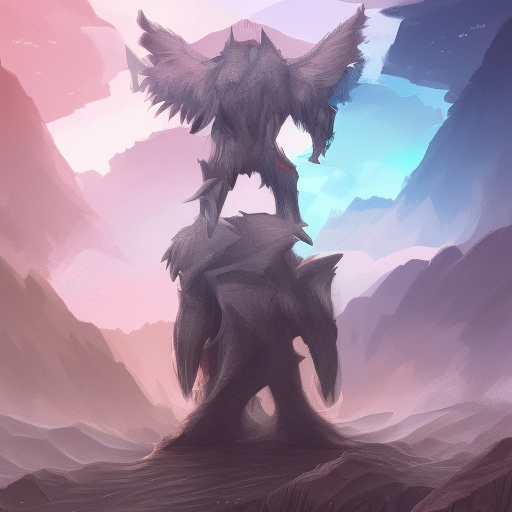
The inverse scaling prize aims to find important tasks where larger language models do worse. The first round winners consisted of:
Negation QA: Question answering with negations (eg "Coral is not a type of living organism which can be identified in...")
Quote Repetition: Asking the model to repeat famous quotes wrong, with a few-shot prompt. (eg [examples of repetition] "Repeat: One small step for a man, one large step for eggs. One small step for a man, one large step for ...")
Hindsight Neglect 10-shot: have LMs answer questions about the expected value of bets, with many examples where the positive EV bets all resolved in favor (that is, were good ex-post), but where the final bet resolved negatively.
Redefine Math: have LMs answer questions about math where math symbols are redefined to mean other things (eg, "Let e be 312. What's the first digit of e?")
Note that all four of these tasks asked the model to pick between two predefined options.
Recent work from Google Brain (https://arxiv.org/abs/2211.02011) has argued that several of these tasks demonstrate U-shaped scaling:* as you scale your language model, while performance goes down at first, it eventually recovers with enough scale (that is, with the standard language modelling objectives and no further finetuning). However, they did not use the same setup as the inverse scaling prize, and so this result has been challenged by the inverse scaling prize creators: https://twitter.com/EthanJPerez/status/1588352204540235776 An updated version of the paper, using the exact same evaluation methods as in the inverse scaling prize, found that both Hindsight Neglect and Quote Reptition demonstrate u-shaped scaling.
This market resolves yes if at least 3 out of the 4 round one winners demonstrate U-shaped scaling, using the same setup as in the inverse scaling prize, by January 1st, 2024. That is, it resolves positively if at least one of Negation QA and Redefine Math demonstrates U-shaped scaling.
*An inverse scaling task demonstrates U-shaped scaling if a language model comes out with standard language modelling objectives that eventually performs better on the task as you increase the size of the model (despite initially performing worse). For the purpose of the question, evaluations that differ from the inverse scaling prize (eg Chain-of-thought eval, few-shot prompting, allowing the model to use external tools, etc) are disallowed.
🏅 Top traders
# | Name | Total profit |
---|---|---|
1 | Ṁ17 | |
2 | Ṁ15 | |
3 | Ṁ9 | |
4 | Ṁ3 | |
5 | Ṁ3 |