People are also trading
Multimodal Contrastive Learning with LIMoE: the Language-Image Mixture of Experts
https://arxiv.org/pdf/2206.02770.pdf
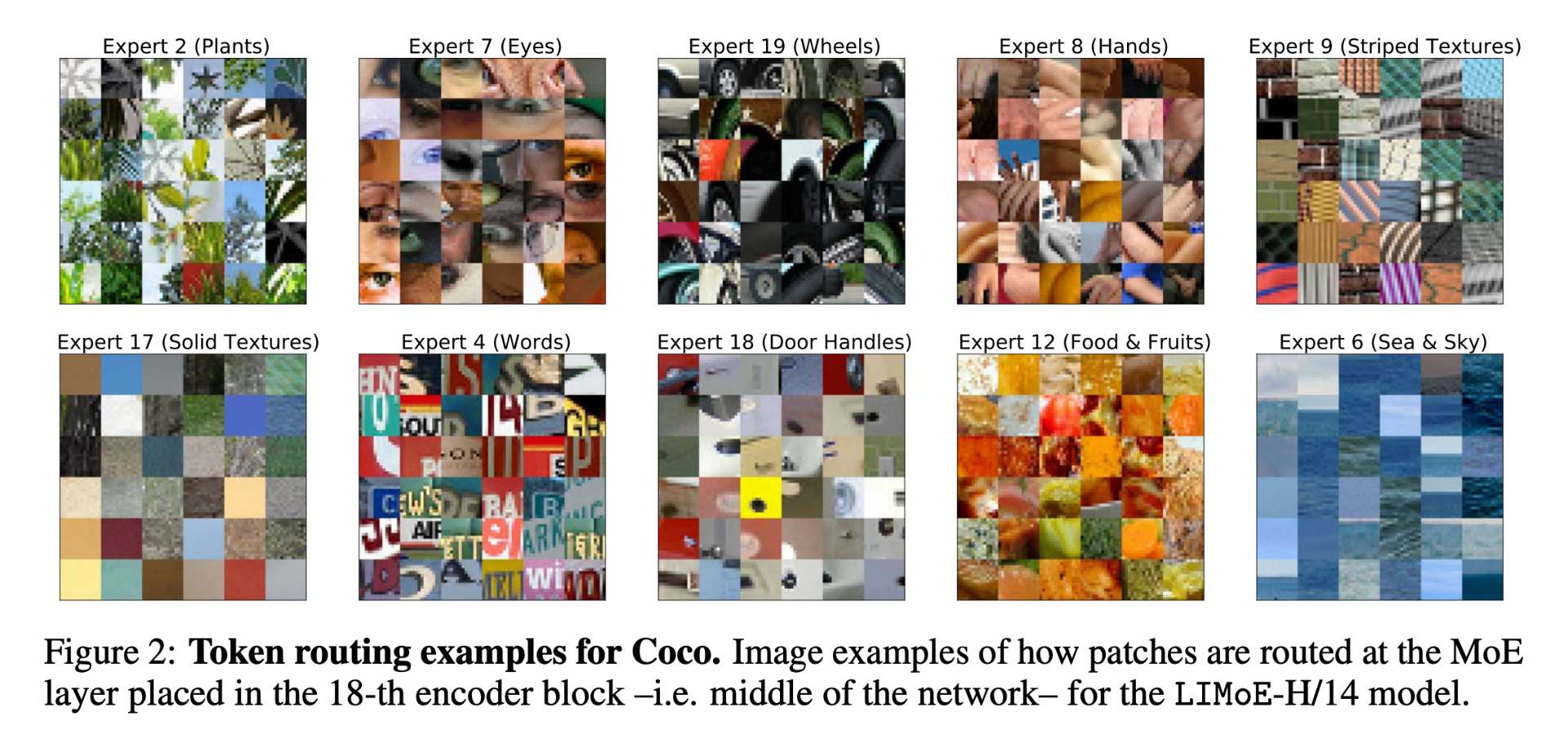
This is using something like top-k so it's not that interesting
@NoaNabeshima To be clear, a lot of my YES weight is on worlds where experts don't cleanly specialize and yet MoE models are still more interpretable.
@NoaNabeshima One consideration is that if we’re controlling for performance, MoE models would have more parameters.
@JSD But I’m still a yes overall - even accounting for this, I don’t expect MoE models to be less interpretable. But I don’t expect MoE’s extra interpretability to be particularly actionable/useful?
@NoaNabeshima For whatever application people have in mind for interp (latent advex, steering via activations, full reverse engineering, mechanistic anomaly detection), I don’t think people working on that application will say things like "phew, thank god we’re working with MoEs", or "if only we were working with MoEs".
Stella’s response is interesting (https://www.lesswrong.com/posts/umkzHoeH2SJ2EcH6K/are-mixture-of-experts-transformers-more-interpretable-than?commentId=k6xkyzbSXCdkhhhrJ), but what if experts are trained on different datasets, which rumors suggest was the case for GPT-4?
@JSD yep, I commented there before making this market. Its possible that experts were trained on different datasets, its interesting.
@JSD I mean I'd guess multi-headed attention is also more interpretable than single-headed attention.