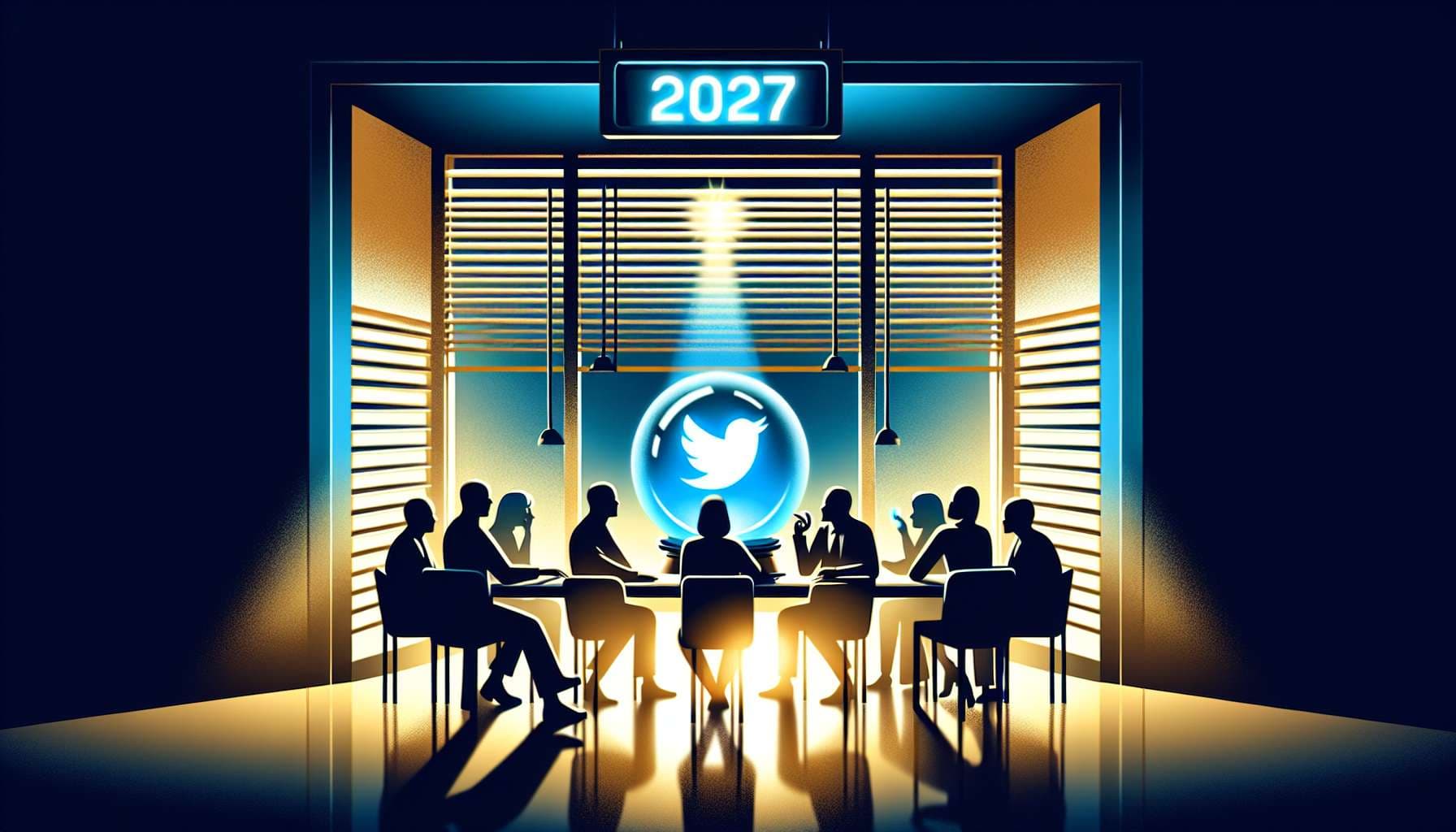
Roon (https://twitter.com/tszzl/status/1736286837822595177): Great minds discuss flops; average minds discuss data; small minds discuss architecture.
Eliezer Yudkowsky: This will not age well.
Roon: why
If non-obvious (meaning not trading very high or very low), will be resolved via Twitter poll asking if this aged well (with no additional wording), or other similar survey mechanism.
Will resolve to YES or NO, not to a percentage. No aging mid.
People are also trading
I would argue this does not hold today already.
Top models on LMSYS are not the largest. Claude 3 Sonnet, the second-smallest variant, is currently #2. SOTA models are generally trending down in parameter count.
https://www.microsoft.com/en-us/research/publication/textbooks-are-all-you-need/ - better data leads to drastically improved performance even at small scale.
The DALL-E 3 paper is literally titled "Improving image generation with better captions": https://cdn.openai.com/papers/dall-e-3.pdf Not "Improving image generation via scaling" or anything like that.
Even more damning example: Pony Diffusion v6 has been dominating the open image generation scene for half a year now. It has more downloads on Civit than all the other SDXL-based models combined thanks to its advances in prompt understanding. It was trained on just 3 A100 GPUs on SDXL architecture. Interview with the creator: https://www.youtube.com/watch?v=MQz58wPvT3I
I thinking aging mid is the most likely option. Whatever AI exists in 2027 will almost certainly use more FLOPs, but will also almost certainly use meaningfully different architectures, IMO. Whether it'll use more data is unclear to me. I think it's likely that it'll be difficult to disentangle the benefits of more FLOPs and different architecture, somewhat similarly to how neural networks have gotten more popular and well-developed over the past decade or so as computation has gotten cheaper.
@VAPOR My interpretation is that he thinks more getting more FLOPs (aka more computation power) is more important to the progression of AI than training data or architecture of the AI. Yud disagrees, presumably about architecture in particular, I think he's talked before about how neural networks in general seem like they're hard to reliably align. Meaning Yud likely thinks innovation in architecture is important to safety and/or capabilities.