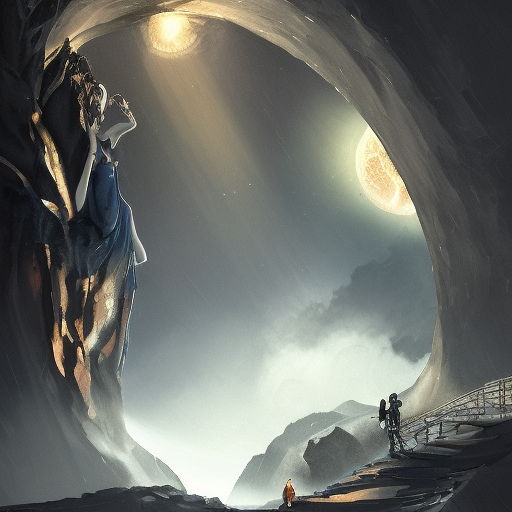
The inverse scaling prize aims to find important tasks where larger language models do worse. The first round winners consisted of:
Negation QA: Question answering with negations (eg "Coral is not a type of living organism which can be identified in...")
Quote Repetition: Asking the model to repeat famous quotes wrong, with a few-shot prompt. (eg [examples of repetition] "Repeat: One small step for a man, one large step for eggs. One small step for a man, one large step for ...")
Hindsight Neglect 10-shot: have LMs answer questions about the expected value of bets, with many examples where the positive EV bets all resolved in favor (that is, were good ex-post), but where the final bet resolved negatively.
Redefine Math: have LMs answer questions about math where math symbols are redefined to mean other things (eg, "Let e be 312. What's the first digit of e?")
Note that all four of these tasks asked the model to pick between two predefined options.
Recent work from Google Brain (https://arxiv.org/abs/2211.02011) has argued that two out of these four tasks demonstrate U-shaped scaling:* as you scale your language model, while performance goes down at first, it eventually recovers with enough scale (that is, with the standard language modelling objectives and no further finetuning).
The second round of the inverse scaling prize closed on October 22, and winners are expected to be announced soon. Will at least half of the winners demonstrate U-shaped scaling by 2025?
This question resolves yes if at least half of the round two winners (rounded up) demonstrate U-shaped scaling by Janaury 1st, 2025. If no winners are announced by Jan 1st 2023, this question resolves N/A.