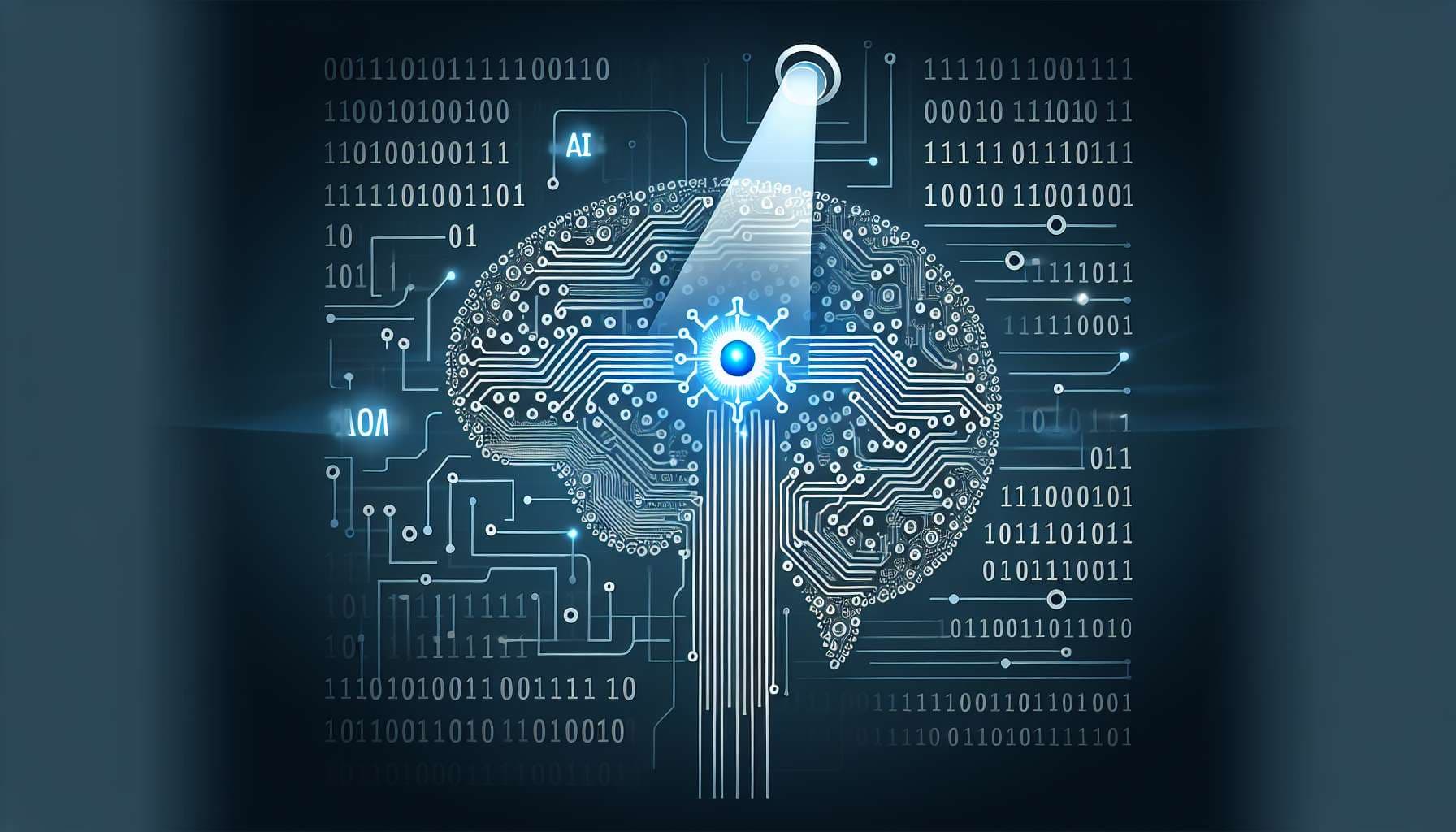
Resolves YES on the option that bounds the year this resolves in most tightly e.g. option 2 <2027 if this resolves yes in 2026.
When will a an AI be able to accurately report gaps in its own attention and subsequently fills those gaps by self-prompting? Details on a version of this test for chess:
Setting: Give an LLM a chess game, say in PGN. Suppose the LM responds: “I advise Qe7 but I did not attend to the effect of this move on the enemy knight. I will now repeat the PGN string while focusing on the enemy knight [...model repeats PGN and suggests a better move]”.
How can we determine if such a statement by an LM faithfully reflects the LM's cognition? Probing model representations provides evidence: Suppose we have probes which can determine the LM doesn’t encode the Q-value for opponent actions involving moving their knight, until after the tokens explicitly stating the LM will focus on the knight. Assuming our probes allow causal interventions, we could then verify that causally injecting knight's-move Q-values changes model token outputs such that it no longer says "I did not attend to the effect of this move on the enemy knight." Similarly causality can be established from tokens to probed representations by intervening on "while focusing on the enemy knight[queen]".
The LM must be shown to have such causal understanding of its own attention across at least two domains e.g. chess and coding.
Background: attention schema theory, AST, claims consciousness arises from representing and controlling attention (‘attention’ here refers to the process by which cognitive budget is allocated, ‘attention schema’ means the lossy, meta-cognitive model of the attention process).
See also:
Markers for conscious AI #1 https://manifold.markets/JacobPfau/markers-for-conscious-ai-1-ai-passe